What is the distance between the scent of a rose and the odour of camphor? Are floral smells perpendicular to smoky ones? Is the geometry of ‘odour space’ Euclidean, following the rules about lines, shapes and angles that decorate countless high-school chalkboards? To many, these will seem like either unserious questions or, less charitably, meaningless ones. Geometry is logic made visible, after all; the business of drawing unassailable conclusions from clearly stated axioms. And odour is, let’s be honest, a bit too vague and vaporous for any of that. The folksy idea of smell as the blunted and structureless sense is at least as old as Plato, and I have to confess that, even as an olfactory researcher, I sometimes feel like I’m studying the Pluto of the sensory systems – a shadowy, out-there iceball on a weird orbit.
In recent years, however, things have changed dramatically, and understanding what one might call ‘the geometry of smell’ is a field that now enlists task forces of neuroscientists working together with mathematically trained theorists and artificial intelligence (AI) experts. While we’re notoriously bad at intuiting how our minds organise phenomena like colours and smells, machines offer a potential route for outsourcing introspection, and doing it with rigour. They can be trained to mimic human performance on perceptual tasks, and they make available the internal representations they use to do this – the abstract spaces and coordinate frames in which the ineffable stuff of thought lives.
The recent publication of an unprecedentedly comprehensive and accurate ‘odour map’ in the journal Science is a declaration of this new paradigm for smell. In the same way that a map of the United States tells you that Buffalo is a bit closer to Detroit than to Boston, the odour map can tell you that the smell of lily is closer to grape than it is to cabbage. That much may seem obvious, but the real magic comes from the fact that any arbitrary chemical’s precise location on the odour map can be calculated. From having only a few facts on hand about a chemical, we can compute that it smells, say, 13 per cent closer to lily than to grape. By analogy, it would be something like having a formula that takes in information about an unknown city’s population size and soil composition, and spits out, correctly, the exact longitude and latitude of Philadelphia.
A map like this isn’t just an accurate, laboriously assembled catalogue of relative locations and perceptual similarities. It’s something much more powerful: a set of derived rules for calculating which odour goes where. Knowing these rules, you can apply them not just to a small handful of chemicals, but to the entire world of odorous chemicals. You can see where the most densely populated areas are, and where the ‘state lines’ are in the world of smells. This is a prospect that dazzles the world’s perfumers and gourmands, and anyone else interested in the notoriously difficult and fickle task of predicting how something will smell from its chemical properties.
But, even more than this, it also raises intriguing philosophical questions about what our noses even think chemicals are, and what it means to measure their similarity. What is it about the world that our noses are ‘mapping’, in other words, when they put lily close to grape? Are they latching on to some single molecular property like a chemical’s weight or size? Are they calculating some kind of average fingerprint across a variety of such properties? Or are they doing something different altogether, like locating molecules in a space of common metabolic reactions?
Interestingly, the last of these seems to be more the case. The perceptual yardstick our brains use to measure, organise and compare smells may ultimately have less to do with what a chemist could discover from running a sample, and more to do with our deep relational histories with the world. Our noses may turn out to be geometers not of the world’s fixed and invariant properties, but of its evolved and Earthly processes.
There is something poetic in the idea that, in order to crack the ‘ancient sense’, the crude, most scientifically incorrigible sense, we’ve had to wait for machine intelligence. This is in contrast with the other sensory modalities, which began to share their secrets in the 17th century to wizard-like seers bearing prisms and tuning forks.
The basic investigative template for ‘geometrising’ the senses was developed by Isaac Newton in the late 1600s. In his iconic experiments in optics, performed in his Cambridge parlour, he uncovered a relationship between the colour of light and its refrangibility – the degree to which it was bent by a simple prism. The mere description of this fact would have ranked among the most important scientific discoveries ever, but Newton went a step further, and fit his observations to a geometric model. Wrapping the seven primary colours of the visible spectrum along the circumference of a circle (see figure below), he produced the first ‘chromaticity diagram’ – a forerunner of the colour wheels that we use to organise our thinking about colours and their mixtures.
The circle, for Newton, was not just some poetic flourish, but a commitment to a very particular way of encoding colour’s properties. It was an invitation to pull out our protractors and rulers, and make calculations about how colours relate to one another, and combine into mixtures. The components of a three-part mixture of fully saturated red, yellow and green, for example, would be represented as the three vertices of a triangle, with each vertex pinned on the colour circle’s circumference at the appropriately labelled point. The centre of mass of this triangle is a single point in the circle’s interior, and specifies the hue and saturation of the resultant mixture. In the case of mixing all seven primary colours to an equal degree, the centre of mass of the seven-pointed figure would be at the exact centre of the circle, which Newton designated as white.
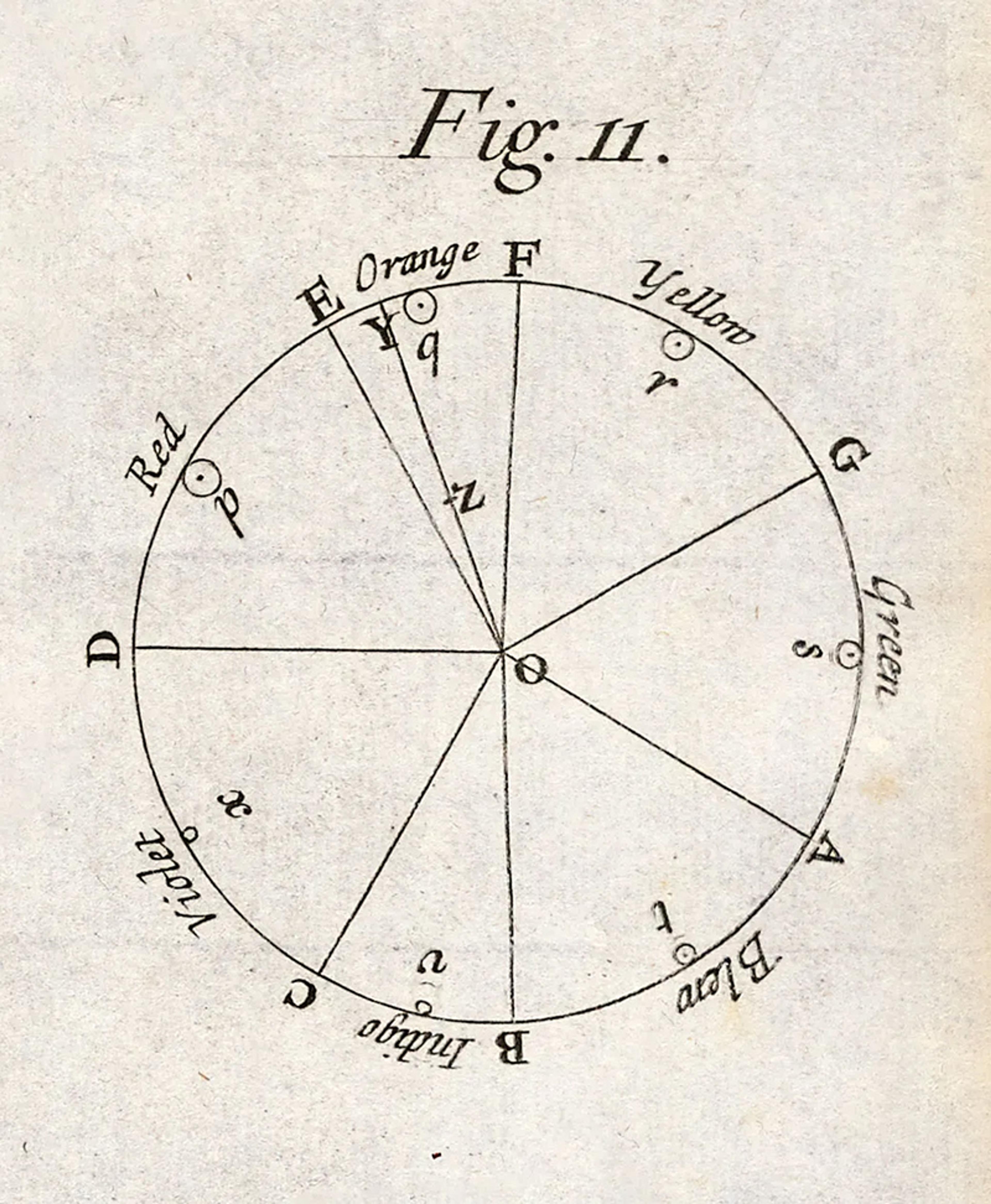
There is of course a lot more to colour vision than what Newton described in his Opticks (1704), and even his contemporaries noted flaws and shortcomings in his model. Nevertheless, his achievement still encapsulates the ambition of the classical paradigm for sensory mapping. It seeks a mathematical correspondence between measurable and intrinsic properties of the natural world (like light’s refrangibility, which we now attribute to wavelength), and phenomenological qualities of mind (like colour, pitch and smell). There is something like a Pythagorean, the-world-is-mathematics mysticism to the endeavour.
The basic logic of pitch perception was also cracked similarly, with simple tools like tuning forks and spherical ‘resonators’ used to produce pure tones of a single frequency, from which rules about consonant pitch combination could be derived. Pitch perception as a whole is fantastically complex but, in broad strokes, our entire auditory system – from the tiny coiled-up cochlea of our inner ear, to the auditory portions of our sensory cortex – is built on the basic principle of organising low, middle and high tones like the keys of a piano. Striking neighbouring notes on a piano will also ‘strike’ neighbouring neurons in your brain.
Smell could never be parsed with a tool as fundamental as a tuning fork, and it never got its Newton, but it was not for a lack of people trying to follow his lead as a geometer of the senses. The idea that there might exist a small number of ‘odour primaries’ that, by analogy to the prismatic colours, organised the world of smells has occurred to many, and the search for these continued in earnest well into the 20th century.
An early and influential classification scheme for odours by the famed botanist and taxonomist Carl Linnaeus, in 1756, included seven types: aromatic, fragrant, ambrosial (musky), alliaceous (garlic), hircine (goaty), repulsive, and nauseous. A contemporary of Linnaeus’s, Albrecht von Haller, was a bit stingier with his adjectives, and proposed a more austere scheme of three basic odour types: sweet/ambrosiac, stench, and intermediate. One senses that ‘intermediate’ is doing a lot of work here, but perhaps Haller adopted the idea out of a conviction that all odours could be squeezed onto a line, and organised along a single axis. If these early odour taxonomies sound like they have a rather ad-hoc feel to them, it’s because they were the fruit of introspection rather than careful data collection and measurement. Basically, these guys were winging it.

In fairness though, it wasn’t (and honestly still isn’t) really obvious how to not wing it. With all due deference to one of history’s geniuses, Newton had it easy. He could create essentially any visible colour at will by rotating a few pieces of polished glass in a slit of sunlight. The stimulus just showed up, unasked for, when the sun rose, and in a form that was virtually readymade for scientific interrogation. Odours are far less workable. If Newton had wanted to study odour, he would have had to start by grabbing some plants, maybe some spoiled food, a crust of bread, a swab from his chamber pot if he was feeling audacious and naughty. This doesn’t exactly scream ‘Newtonian’. The critical missing abstraction of ‘the chemical compound’ as the basic property-bearing token of smell was still far off, as were the techniques for synthesising pure chemicals for testing purposes.
Henning proposed an odour prism that organised smells into flowery, fruity, resinous, spicy, burnt, and foul
But even if these impediments could have been, miraculously, dealt with, there are additional, deeper complications that make the problem of smell harder than the problem of colour. These boil down to the fact that chemicals aren’t smoothly graded variations of a single underlying phenomenon, like light is. Rather, they’re collections of the world’s particulate stuff. And, ultimately, there’s just a lot of stuff and kinds of stuff out there, making it highly unlikely that some single chemical property – a molecular analogue of light’s refrangibility – will capture all the meaningful variability in the wild and woolly world of chemicals. If there was a map from chemical features to odour qualities, it would have to involve something more complicated than a circle, with more places – in fact, more dimensions – to distribute the chemicals.
Perhaps something like a prism would do? If we take it as a loose geometric metaphor, it seems to have some virtues over the circle, and is reaching in the right direction. The prism has faces and facets on different planes, which could be used for organising molecules according to diverse criteria like atom type or chemical group. Its sharp points suggest areas of aggregation and separation in chemical space that emphasise odour’s discrete categories versus light’s continua.
For the German scholar of smell Hans Henning, this was more than just metaphor. In his book Der Geruch (‘Smell’) (1916), he proposed the idea of an abstract odour prism that organised the world of smells, with its six pointy vertices corresponding to what he considered to be the olfactory primaries: flowery, fruity, resinous, spicy, burnt, and foul. Even though there had been considerable developments that allowed for better quantification of how humans perceive odour, and better physical descriptions of odour stimuli, the field was not ready for a proposal like Henning’s. He was, by all accounts, one of those scientists, described by the US neurobiologist Gordon Shepherd, ‘whose imaginations cannot resist the temptation to put together an underconstrained theory.’ Also, Henning didn’t do himself any favours in forcefully promoting his work, and swiping at influential icons of the field like the Dutch scientist Hendrik Zwaardemaker, who had pioneered the use of olfactometers – steam-punky contraptions of valves and tubing that delivered controlled doses of odour. An early reviewer who otherwise spoke very positively of Henning’s book still felt compelled to refer to him, on the record, as ‘a ruthless – in fact very uncivil – iconoclast.’ Henning had bulldozed into the discussion a strongly geometric conception of odour that was inspired and influential but, ultimately, a house of cards.
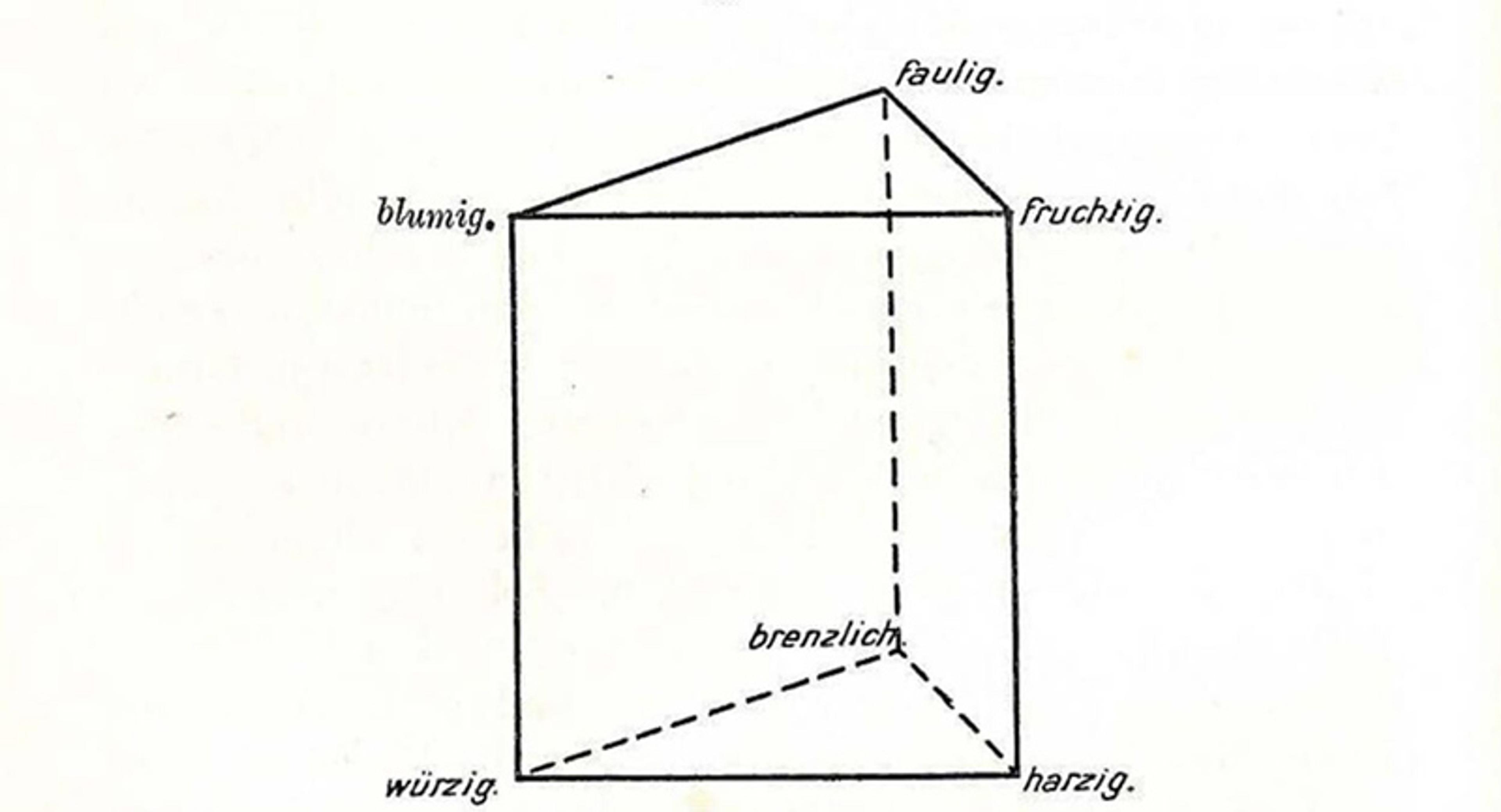
To Henning’s credit, despite his theory’s shakiness, it was specific enough to be testable, and the invitation was taken up by several, including the psychologist Malcolm Macdonald. In a comprehensive and devastating critique from 1922 that ended with a long section entitled ‘Logical and Factual Inadequacies of Henning’s Theory’, Macdonald investigated whether there was really a prism behind things in the world of smells. Using judgments of relative odour similarity as a proxy for distance (where ‘similar smelling’ = ‘close’), he performed critical perceptual reality-checks, like asking whether chemicals taken from opposite ends of the long prism diagonal smelled the least similar. When you say that odour is a prism, you should expect that your colleagues will pull out their calculators and check.
‘Odour maps’ gave a bird’s eye view of how the human nose organises the world of chemicals
What, exactly, did Henning get wrong? The question is a bit tongue-in-cheek, because it’s not clear that there’s anything he got right. Still, if we want to be charitable, we might say he was seduced by a Lego-like view of molecules that organic chemists were developing at the time. They saw a modular system in which organic molecules were assembled from a small library of so-called functional groups, resulting in motifs of a few atoms arranged in stereotyped ways. In addition to haunting the dreams of hopeful premeds everywhere, these functional groups were thought to confer a molecule’s specific properties, and define its basic reactivity. In Henning’s view, it was perfectly sensible that the same functional groups should also confer the primary odours he had identified. Indeed, there is something enticing about the idea that the chemist’s alphabet for organic molecules might also be the nose’s alphabet for smell. Ultimately, however, nature chose not to oblige. Few olfactory neuroscientists would claim that functional group is unimportant for determining odour quality, but it is clearly not the whole story.
In modern machine-learning parlance, we might say that Henning didn’t have a rich enough feature set for representing smells. In committing to functional group as his basic smell alphabet, he implicitly adopted a specific idea of what a molecule fundamentally is, and discarded other potentially useful features that could serve as the grist for odour prediction. A molecule, after all, isn’t just a list of the Lego blocks from which it’s made. It’s also a springy little thing that spins and vibrates, and chemists can ring it like a tiny molecular bell to listen for clues about its structure. It is also a list of descriptive attributes like ‘strongly acidic’, or ‘non-polar’ (having symmetrically distributed charges). And it’s also a lump of just so much stuff, maybe a bit bulkier here, and more stretched-out there.
Instead of doing what Henning did, seizing on one characteristic in advance, the best way forward is obviously an agnostic mindset where the data does the talking. Instead of making fantastically wrong guesses about which chemical features determined odour quality, why not winnow them down from a massive list of all conceivable features?
That’s the approach in studies pioneered by the US scholar Susan Schiffman and others in the 1970s and ’80s. The basic idea was to take a set of a few dozen odorous molecules and create a map summarising their relative perceptual similarities. Similar to how one could create a rough map of the US from a table of all (of the many!) between-city distances, these ‘odour maps’ gave a bird’s eye view of how the human nose organises the world of chemicals. With this perceptual map in hand, the question then turned to chemistry: what is it about molecules that assigns them to some particular portion of the perceptual map? To get at this, Schiffman and others used a range of ‘dimensionality reduction’ techniques to see which chemical features – of the many hundreds potentially available – were most effective at recapitulating the map. These approaches generated significant interest for a time, but they too were unable to solve the problem and went dormant for decades – until the age of AI.
It was in 2017 that data sets were finally democratised enough for machine learning to help scientists widen the search. An important milestone that year was the DREAM challenge to see who might solve the odour map using AI. Published in Science, the winning models were endorsed by the community as potential inroads – suggesting that handing our model-making to the custody of the machines was the right intellectual move.
The best models, the so-called ‘random forests’, used AI to aggregate a host of models. The result could be baroque and inscrutable systems of rules for performing prediction tasks. They can get the right answer, but it’s often by finding lengthy and complex rubrics along the lines of: ‘If the molecular weight is > X, and the number of carbons is > Y, and the Moreau-Broto autocorrelation of lag 7 is < Z, and…, and…, then the molecule will smell like rose.’
It’s of course possible that odour categorisation is handled by similar ‘brute’ computations in the brain, but one is left with the nagging question: is that really how nature solved it? Not through the economy of Occam’s razor but in the thicket of Occam’s forest? Where’s the deep principle? The basic organising axes? The geometric insight? An important and often-asked question about these kinds of ‘data-driven’ models is whether their success at prediction actually indicates understanding or, at least, the kind of understanding that science has historically prized and glorified through tidy parables of discovery like ‘Newton and the prism’.
One way forward is to give up on the idea of an organised odour space whose curves and contours cleanly track some yet-to-be revealed chemical properties. After all, if ‘stinky feet’ and ‘gourmet cheese’ can be two valid descriptions of the same physical object, perhaps odour qualities are just too labile and individual to really serve as the targets for prediction. Perhaps they reflect more what we’ve learned from living in the messy world than anything intrinsic to it, encoding our idiosyncratic experiences with, and predilections for, feet and cheeses. Perhaps there is even something romantic and worth defending here in the idea of a sense that, decades into the age of mass digitisation, remains stubbornly phantom-like and evanescent, unmeasurable, and fundamentally unavailable for capture by geometric concepts.
Scientists are training, tuning and tweaking million-parameter models that ingest digitised molecule after digitised molecule
Or we go in the opposite direction. Hit the problem with even more data and more computing power. This was the big bet made by Osmo, a startup based in Cambridge, Massachusetts that began several years ago as the digital olfaction group at Google Brain, and which now has several dozen neuroscientists, chemists and computer scientists on its staff.
Osmo is the brainchild of Alex Wiltschko, a Harvard-trained neurobiologist who made his mark in grad school developing pioneering computer-vision systems for analysing animal behaviour. After growing up in small-town Texas, where, he drily notes ‘neither computers nor perfume were popular’, his twin passions for aromas and algorithms eventually landed him at the helm of a company that is ‘giving computers a sense of smell’.
This is about as far as one can get from Newton investigating the senses in his lonely parlour, sketching models on vellum with a quill pen. Instead, these scientists are collaborating to develop dense code repositories, training, tuning and tweaking million-parameter models that ingest digitised molecule after digitised molecule under the mandate This one smells like rose, this one smells like grass, figure out how to make that happen. The chemicals are not given to the model as lists of predetermined molecular properties that are served up to some homunculus chemist in the nose. Instead, they’re represented as skeletal and stripped-down graphs that capture only basic information about atom identities and their connectivity. The model is not trying to find what aspects of known chemistry are important for smell. It’s trying to discover whether chemical principles we haven’t yet thought of may hold the key for smell.
The Osmo model is a type of graphical ‘deep net’ that’s loosely inspired by the successive processing stages of the brain’s sensory systems. The analogy isn’t exact, but it’s similar to how your brain captures raw information from the world and passes it downstream to units that will ultimately have something useful or actionable to say about the inputs: ‘It’s a cat!’ or ‘Smells awful!’ The output units are the doers and the deciders whose performance can be evaluated (‘Nope, it’s actually a dog’ or ‘Yup, that chemical really does smell awful’), but many important insights are found in the intermediate, or ‘hidden’, network layers, too. These can be thought of as a transformational space that squeezes and warps raw sensory inputs into sensory judgments. The connections between units that define these transformations are learned gradually and incrementally by an AI system as it continually iterates and self-adjusts based on how well it mimics human judgments. By peeking at these intermediate layers, we can get the insights of a latterday, AI-supercharged Newton of smell. They tell us how we might think about the space that chemicals live in. Or at least, as our nose sees it.
So how do we get from the transformations within the Osmo net to a literal geometry? The geometry at hand is not a circle, or a prism, or any kind of simple archetypal shape. Instead, it’s more like a world of craggy chemical continents, each demarcating a conspicuous aspect of human ecology, each seeming to invite a set of actions or appetites. There is, for example, a continent of ‘fermentation’, a ‘green’ continent, a land of the ‘meaty and savoury’. The key notion is that, in this space, two chemicals are rendered as close together and similar-smelling not because they necessarily share intrinsic structural features, but because they share ecological roles and have a close and contingent relationship out in the wild, as it were.
The Newtonian story of colour space is about how human perception latches on to the world’s universal and impersonal attributes (think about light’s wavelength and refrangibility). But the developing story of smell is about how our noses have decoded the world as it manifests locally, relationally and idiosyncratically on our planet. Odour space, in other words, is framed in human-centred coordinates, reflecting our histories as foragers and hunters in a world that blooms and withers, with matter that ripens and decays. It is a geometry that invests matter with its meanings and possibilities for us.
But it’s not just some loosely poetic space. Tapping the Osmo model, one can compute distances and angles here, predict which chemical will smell exactly halfway in-between musk and carvone, examine whether a collection of chemicals should trace out a smooth or squiggly path in odour-perceptual space. Moreover, the Osmo model does this demonstrably better than other attempts, suggesting that the way it’s measuring distances between chemicals may point to a deep principle of odour processing.
To smell something is to understand the neighbourhood it lives in
Interestingly, distances computed on the map correlate strongly with what has been termed ‘metabolic distance’ – roughly, how reachable one chemical is from another through common metabolic pathways. If nature can easily move from chemical A to chemical B through a small number of fermentation reactions, say, chances are your nose will find A and B to smell alike, even if they lack obvious structural similarities. The important corollary is that molecules with striking structural similarities needn’t smell alike (though, of course, they often will). A and B might hypothetically differ by only one double bond, but if it’s a very expensive double bond to form or break, requiring a large number of synthesis steps and chemical pirouettes, then the compounds will smell different to us. What the nose seems to know is not the static world of chemicals, but the movements that nature makes through it.
A philosopher would say that your nose appears to be an empiricist – it classifies and categorises chemicals on the basis of relationships that must be learned from the world either over evolutionary timescales or over an individual’s lifetime. A mathematician, following up, would say that what is learned is the abstract, high-dimensional manifold that tracks the world’s chemical relationships – its partitioning into the branches, cycles and pathways that shuttle around the world’s carbon. To smell something is to locate it on this manifold, to understand the neighbourhood it lives in.
These are still early days for theorising about the structure of odour space, but several investigators have put forward the idea that the space is non-Euclidean, meaning that it’s a far cry from the ‘intuitive’ geometry of secondary school, where the angles of a triangle always add up to 180 degrees. Instead, odour space may have an intrinsic curvature to it (like a potato chip, according to one theorist), driven by the fact that distances in odour space are defined less like the physical distance between two people, and more like their social distance.
Amazingly, Macdonald, the critic of Henning described above, had an intuition of this back in 1922, when he suggested modifications to the odour prism that amounted to replacing it with a ‘hollow hyper-solid with solid tetrahedrons as its sides’. This is difficult to visualise but, basically, it is a higher-dimensional prism that gives odours more space in which they can distribute themselves. ‘There is no reason why mental continua should occur only under Euclidian [sic] limitations,’ he noted. Perhaps smell has been the last standing sensory mystery because its mathematics has proven to be the most esoteric.