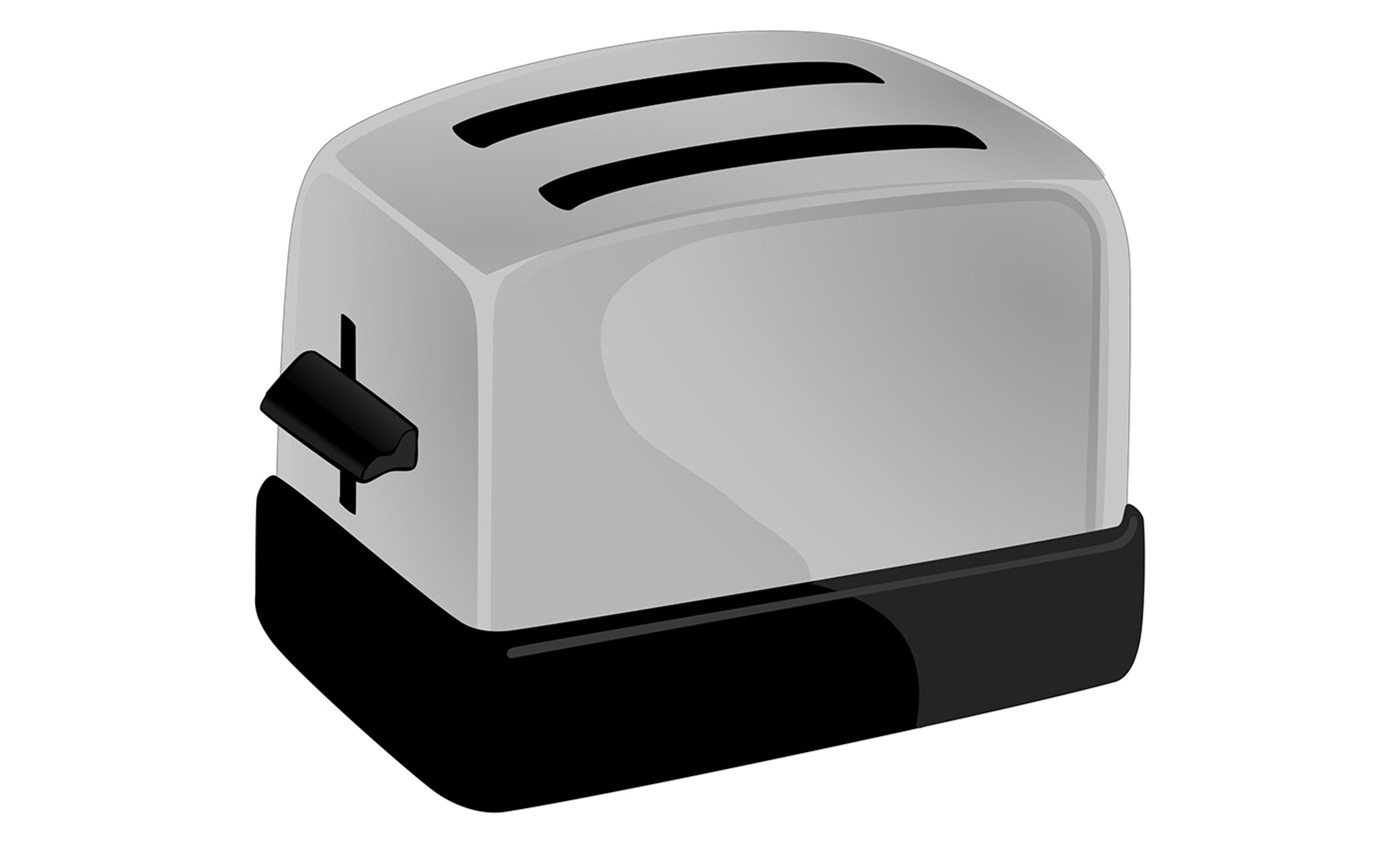
Photo courtesy Public Domain Pictures
Will the intelligent algorithms of the future look like general-purpose robots, as adept at idle banter and reading maps as they are handy in the kitchen? Or will our digital assistants look more like a grab-bag of specialised gadgets – less a single chatty masterchef than a kitchen full of appliances?
If an algorithm tries to do too much, it gets in trouble. The recipe below was generated by an artificial neural network, a type of artificial intelligence (AI) that learns by example. This particular algorithm scrutinised about 30,000 cookbook recipes of all sorts, from soups to pies to barbecues, and then tried to come up with its own. The results are, shall we say, somewhat unorthodox:
Spread Chicken Rice
cheese/eggs, salads, cheese
2 lb hearts, seeded
1 cup shredded fresh mint or raspberry pie
1/2 cup catrimas, grated
1 tablespoon vegetable oil
1 salt
1 pepper
2 1/2 tb sugar, sugar
Combine unleaves, and stir until the mixture is thick. Then add eggs, sugar, honey, and caraway seeds, and cook over low heat. Add the corn syrup, oregano, and rosemary and the white pepper. Put in the cream by heat. Cook add the remaining 1 teaspoon baking powder and salt. Bake at 350F for 2 to 1 hour. Serve hot.
Yield: 6 servings
Now, here’s an example of a recipe generated by the same basic algorithm – but instead of data that included recipes of all sorts, it looked only at cakes. The recipe isn’t perfect, but it’s much, much better than the previous one:
Carrot Cake (Vera Ladies”
cakes, alcohol
1 pkg yellow cake mix
3 cup flour
1 teaspoon baking powder
1 1/2 teaspoon baking soda
1/4 teaspoon salt
1 teaspoon ground cinnamon
1 teaspoon ground ginger
1/2 teaspoon ground cloves
1 teaspoon baking powder
1/2 teaspoon salt
1 teaspoon vanilla
1 egg, room temperature
1 cup sugar
1 teaspoon vanilla
1 cup chopped pecans
Preheat oven to 350 degrees. Grease a 9-inch springform pan.
To make the cake: Beat eggs at high speed until thick and yellow colour and set aside. In a separate bowl, beat the egg whites until stiff. Speed the first like the mixture into the prepared pan and smooth the batter. Bake in the oven for about 40 minutes or until a wooden toothpick inserted into centre comes out clean. Cool in the pan for 10 minutes. Turn out onto a wire rack to cool completely.
Remove the cake from the pan to cool completely. Serve warm.
HereCto Cookbook (1989) From the Kitchen & Hawn inthe Canadian Living
Yield: 16 servings
Sure, when you look at the instructions more closely, it produces only a single baked egg yolk. But it’s still an improvement. When the AI was allowed to specialise, there was simply a lot less to keep track of. It didn’t have to try to figure out when to use chocolate and when to use potatoes, when to bake, or when to simmer. If the first algorithm was trying to be a wonder-box that could produce rice, ice cream and pies, the second algorithm was trying to be something more like a toaster – specialised for just one task.
Developers who train machine-learning algorithms have found that it often makes sense to build toasters rather than wonder-boxes. That might seem counterintuitive, because the AIs of Western science fiction tend to resemble C-3PO in Star Wars or WALL-E in the eponymous film – examples of artificial general intelligence (AGI), automata that can interact with the world like a human, and handle many different tasks. But many companies are invisibly – and successfully – using machine learning to achieve much more limited goals. One algorithm might be a chatbot handling a limited range of basic customer questions about their phone bill. Another might make predictions about what a customer is calling to discuss, displaying these predictions for the human representative who answers the phone. These are examples of artificial narrow intelligence (ANI) – restricted to very narrow functions. On the other hand, Facebook recently retired its ‘M’ chatbot, which never succeeded in its goal of handling hotel reservations, booking theatre tickets, arranging parrot visits, and more.
The reason we have toaster-level ANI instead of WALL-E-level AGI is that any algorithm that tries to generalise gets worse at the various tasks it confronts. For example, here’s an algorithm trained to generate a picture based on a caption. This is its attempt to create a picture from the phrase: ‘this bird is yellow with black on its head and has a very short beak’. When it was trained on a dataset that consisted entirely of birds, it did pretty well (notwithstanding the strange unicorn horn):
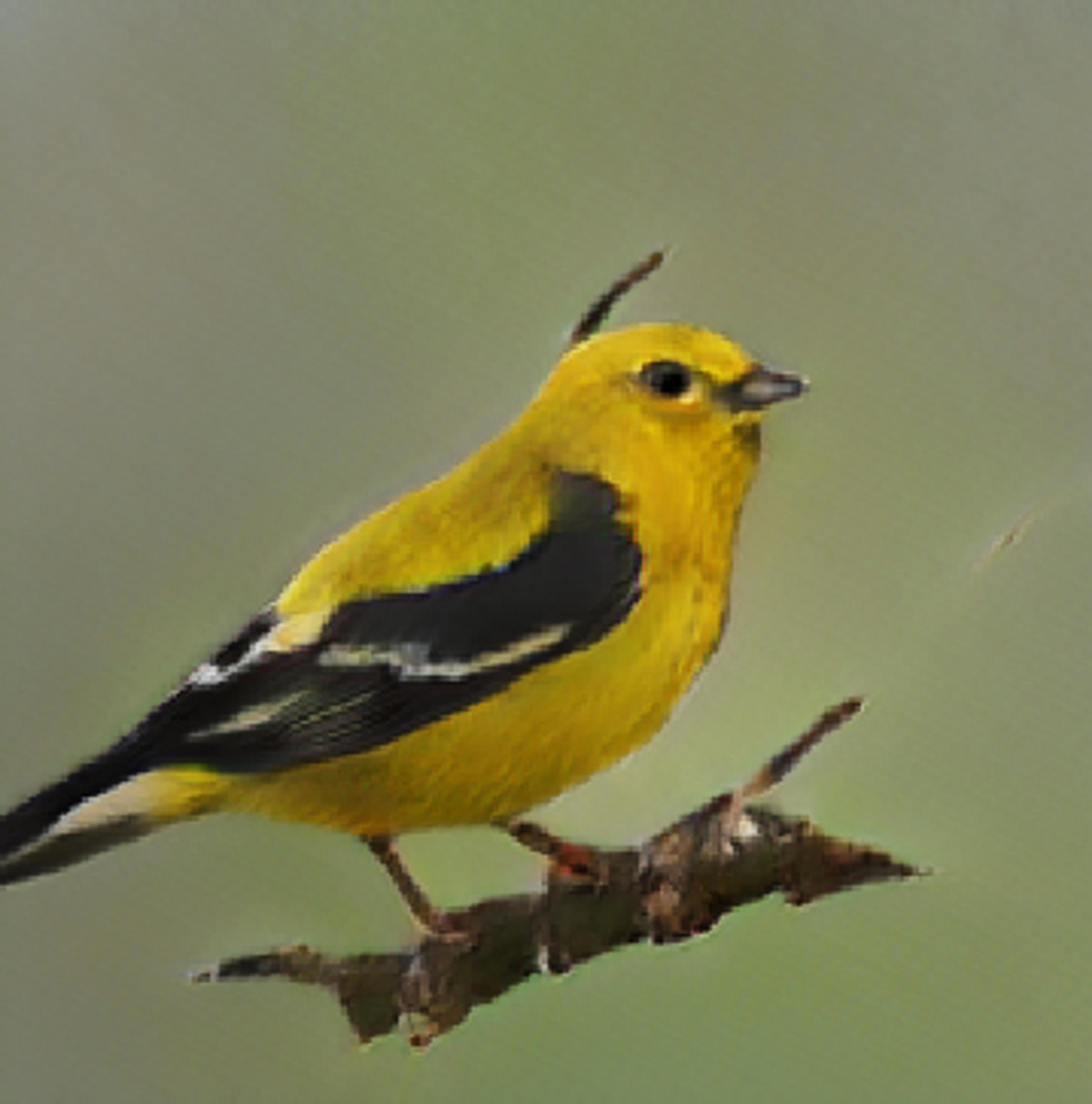
But when its task was to generate anything – from stop signs to boats to cows to people – it struggled. Here is its attempt to generate ‘an image of a girl eating a large slice of pizza’:
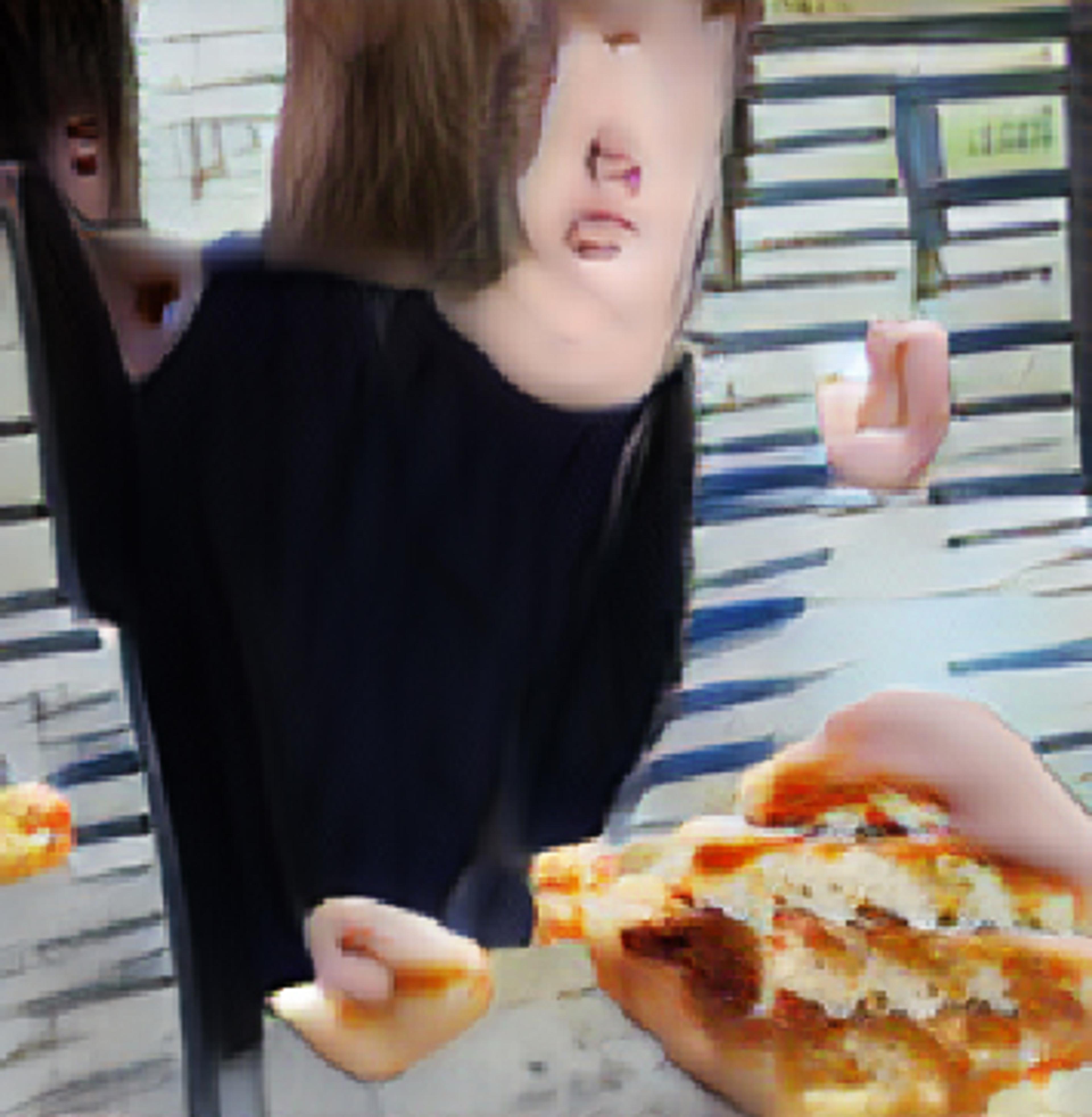
We’re not used to thinking there’s such a huge gap between an algorithm that does one thing well, and an algorithm that does lots of things well. But our present-day algorithms have very limited mental power compared with the human brain, and each new task spreads them thinner. Think of a toaster-sized appliance: it’s easy to build in a couple of slots and some heating coils so it can toast bread. But that leaves little room for anything else. If you try to also add rice-steaming and ice-cream-making functionality, then you’ll have to give up one of the bread slots at least, and it probably won’t be good at anything.
There are tricks that programmers use to get more out of ANI algorithms. One is transfer learning: train an algorithm to do one task, and it can learn to do a different but closely related task after minimal retraining. People use transfer learning to train image-recognition algorithms, for example. An algorithm that has learned to identify animals has already garnered a lot in the way of edge-detecting and texture-analysing skills, which it can move across to the task of identifying fruit. But, if you retrain the algorithm to identify fruit, a phenomenon called catastrophic forgetting means that it will no longer remember how to identify animals.
Another trick that today’s algorithms use is modularity. Rather than a single algorithm that can handle any problem, the AIs of the future are likely to be an assembly of highly specialised instruments. An algorithm that learned to play the video game Doom, for example, had separate, dedicated vision, controller, and memory modules. Interconnected modules can also provide redundancy against failure, and a mechanism for voting on the best solution to a problem based on multiple different approaches. They might also be a way to detect and troubleshoot algorithmic mistakes. It’s normally difficult to figure out how an individual algorithm makes its decisions, but if a decision is made by cooperating sub-algorithms, we can at least look at each sub-algorithm’s output.
When we envision the AIs of the far future, maybe WALL-E and C-3PO aren’t the droids we should be looking for. Instead, we might picture something more like a smartphone full of apps, or a kitchen cupboard filled with gadgets. As we prepare for a world of algorithms, we should make sure we’re not planning for thinking, general-purpose wonder-boxes that might never be built, but instead for highly specialised toasters.