When Galileo looked at the Moon through his new telescope in early 1610, he immediately grasped that the shifting patterns of light and dark were caused by the changing angle of the Sun’s rays on a rough surface. He described something akin to mountain ranges ‘ablaze with the splendour of his beams’, their sides in shadow like ‘the hollows of the Earth’; he also rendered these observations in a series of masterful drawings. Six months before, the English astronomer Thomas Harriot had also turned the viewfinder of his telescope towards the Moon. But where Galileo saw a new world to explore, Harriot’s sketch from July 1609 suggests that he saw a dimpled cow pie. Why was Galileo’s mind so receptive to what lay before his eyes, while Harriot’s vision deserves its mere footnote in history?
Learning to see is not an innate gift; it is an iterative process, always in flux and constituted by the culture in which we find ourselves and the tools we have to hand. Harriot’s 6-power telescope certainly didn’t provide him with the level of detail of Galileo’s 20-power. Yet the historian Samuel Y Edgerton has argued that Harriot’s initial (and literal) lack of vision had more to do with his ignorance of chiaroscuro – a technique from the visual arts first brought to full development by Italian artists in the late 15th century.
By Galileo’s time, the Florentines were masters of perspective, using shapes and shadings on a two-dimensional canvas to evoke three-dimensional bodies in space. Galileo was a friend of artists, and someone who in his youth might have considered becoming one himself. He believed with a kind of religious fervour that the creator of the world was a geometer. Galileo likely imbibed these mathematically deep methods of representation, based as they are on the projective geometries of light rays. Harriot, on the other hand, lived in England, where general knowledge of these representational techniques hadn’t yet arrived. The first book on the mathematics of perspective in English – The Art of Shadows by John Wells – appeared only in 1635. When Galileo looked at the face of the Moon, he had no trouble understanding that lunar mountaintops first catch fire with the rising Sun while their lower slopes remain in darkness, just like they do on Earth. Galileo therefore had a theory for what he was seeing when those pinpricks of light winked into existence along the terminator line of day and night; he even used the effect to measure the heights of those mountains, finding them higher than the Alps. Harriot, a brilliant polymath yet possibly blind to this geometry, looked at the same scenes half a year before Galileo, but didn’t understand.
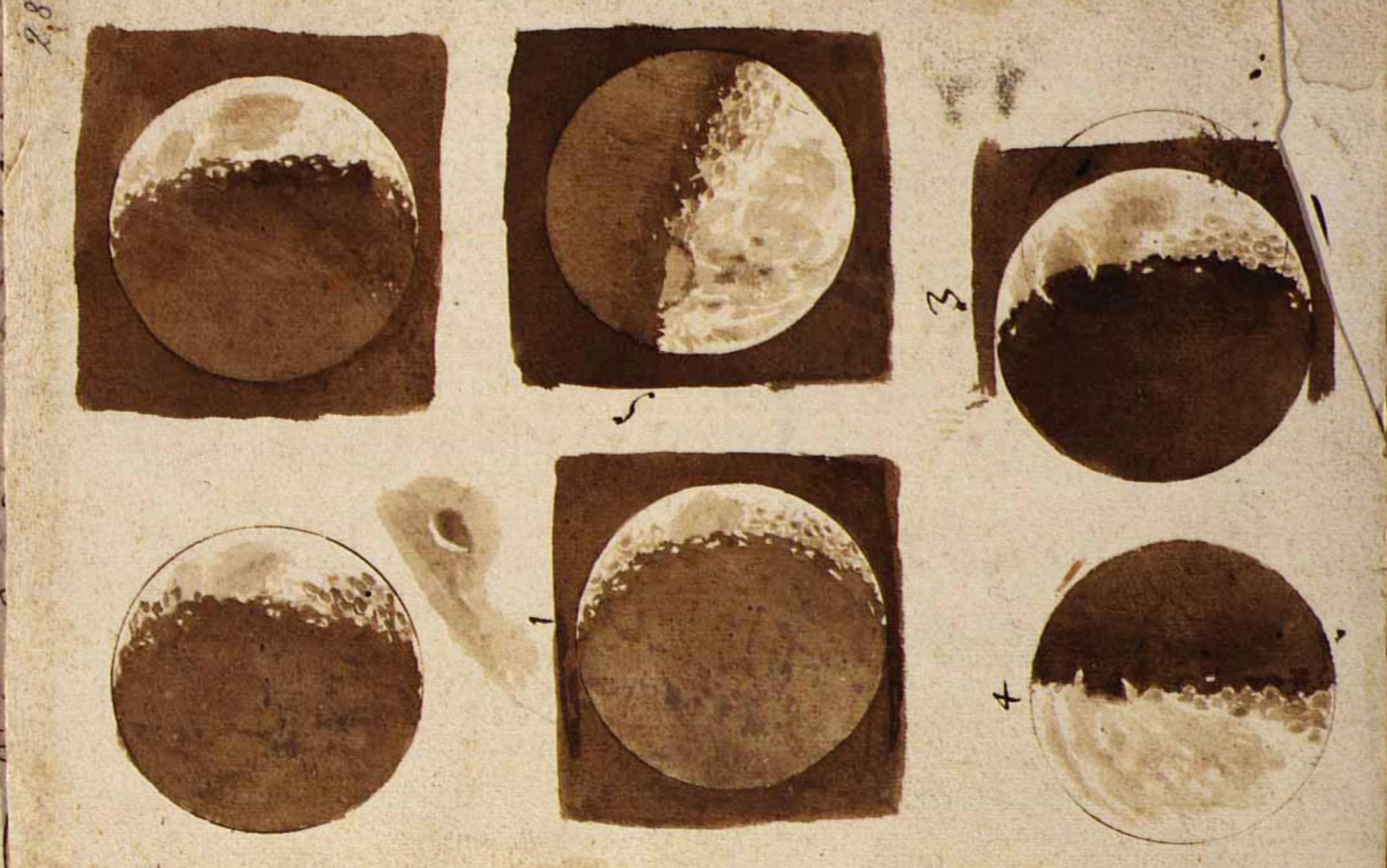
Drawings of the Moon, November-December 1609, Galileo Galilei (1564-1642). Courtesy Wikipedia
When we consider scientific observations – those paragons of a purportedly objective gaze – we find in fact that they are often complex, contingent and distributed phenomena, much like human vision itself. Assemblies of high-powered machines that detect the otherwise undetectable, from gravitational waves in the remotest cosmos to the minute signals produced by spinning nuclei within human cells, rely on many forms of ‘sight’ that are neither simple nor unitary. By exploring vision as a metaphor for scientific observation, and scientific observation as a kind of seeing, we might ask: how does prior knowledge about the world affect what we observe? If prior patterns are essential for making sense of things, how can we avoid falling into well-worn channels of perception? And most importantly, how can we learn to see in genuinely new ways?
Scientific objectivity is the achievement of a shared perspective. It requires what the historian of science Lorraine Daston and her colleagues call ‘idealisation’: the creation of some simplified essence or model of what is to be seen, such as the dendrite in neuroscience, the leaf of a species of plant in botany, or the tuning-fork diagram of galaxies in astronomy. Even today, scientific textbooks often use drawings rather than photographs to illustrate categories for students, because individual examples are almost always idiosyncratic; too large, or too small, or not of a typical colouration. The world is profligate in its variability, and the development of stable scientific categories requires much of that visual richness to be simplified and tamed.
In 1890, the meteorologists Hugo Hildebrandsson, Wladimir Köppen and Georg Neumayer published a most unusual attempt at idealisation. Theirs was the first ‘cloud atlas’, proposing to standardise terminologies and categories of wisps of water vapour. To earlier generations, this had seemed a hopeless project. Clouds are things of infinite variety and shape, a drifting canvas for daydreams. But the creation of agreed-upon names and categories – the feathery high-altitude cirrus, the brooding low-lying stratus, the puff-ball cumulus – this parsing of the visual world overhead and the creation of a shared vocabulary was a great advance of 19th-century meteorology.
Clouds, as it turned out, are a helpful proxy that allow large numbers of semi-skilled observers on the ground to visualise the flow conditions overhead. Following the simple instructions provided by Hildebrandsson and his colleagues, which eventually became an international scientific effort, the observers would know how to report observations of cloud types and the directions of motion, along with local weather conditions. Taken over the course of a year, commencing in May 1896, this compendium of information would allow meteorologists to puzzle out the pattern of winds in the upper atmosphere for the first time.
To say that we construct idealised categories is not to say that patterns in the world don’t already exist
I was interested in seeing the International Cloud Atlas (1896) first-hand. Even though I am a theoretical physicist, I still harbour a need to hold something in my hand, and to see it with my own eyes, before I believe I’ve grasped its nature. While the Atlas has been updated many times, and the current version is available online, there are not many copies of the 1896 Atlas left, so I had to take a road trip to visit the Special Collections Library at the University of Virginia. Instead of the book I expected, the librarian presented me with what looked like an artist’s portfolio, bound by a ribbon: eight pages of text and a collection of 28 ‘photochromotypes’ that were as fragile as old family photographs.
The foregrounds are particularly intriguing as attempts to frame and contextualise forms that would otherwise be free-floating. A water tank pokes into the sky in the photograph of the cirrus; rooflines in Paris are photographed beneath mottled strato-cumulus; a young man dawdles on the bank of a river, in pastel, gazing up at low-lying stratus; and a sailing ship is painted upon a calm ocean, eternally underway beneath a hazy bank of alto-stratus. The need to supplement the photographs with drawings and paintings is an example of idealisation at work. Photographers had only recently learned how to capture the sky in a manner that brought out the shapes of clouds, and so the first Atlas is a mix of photographs and artful renderings in paint and pastel by human hands. The creation of objective categories of cloud-shapes thus involved a collaboration between art and science, driven by a love of shape and form, and the uncovering of an orderly beauty in the sky overhead.
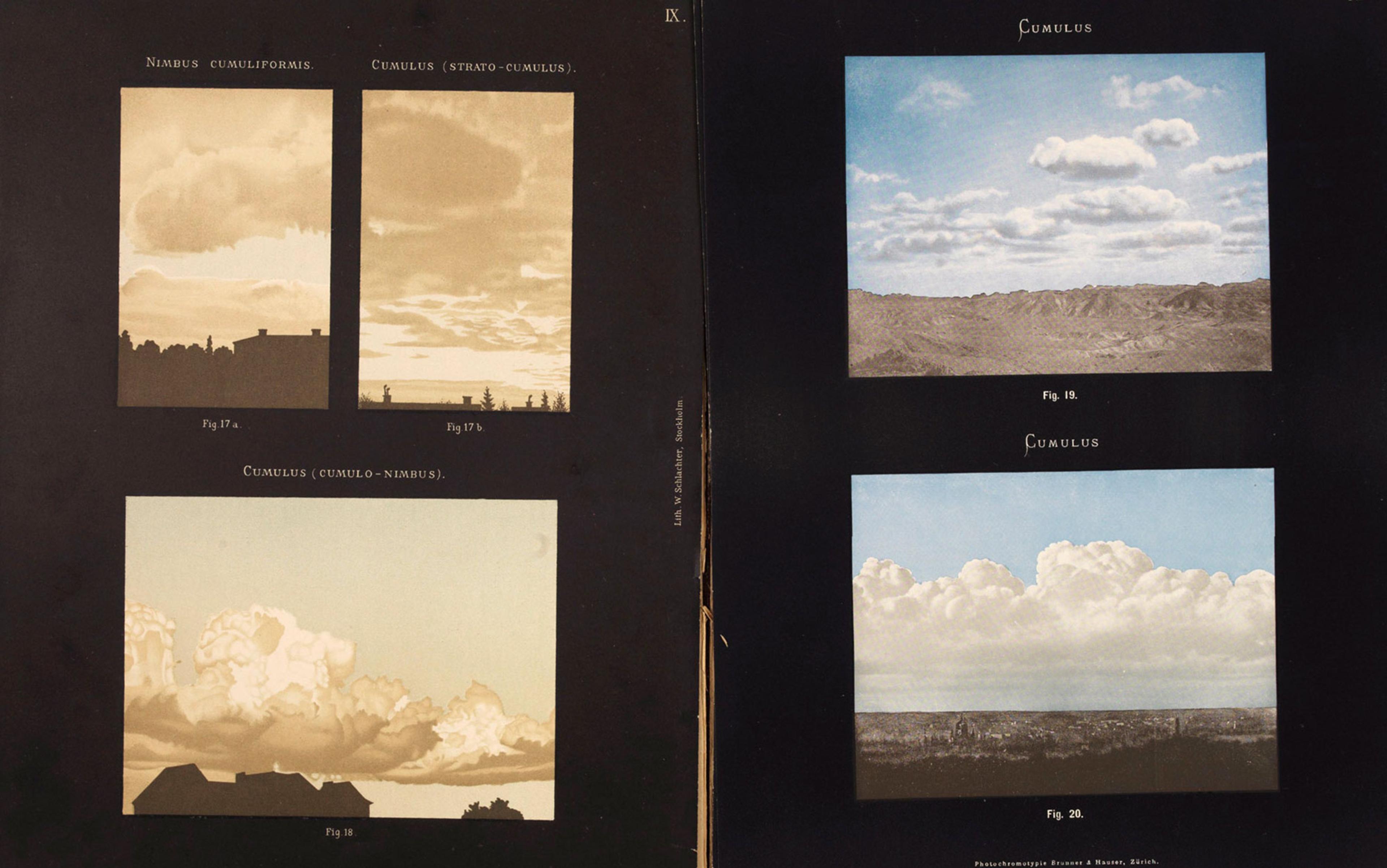
From the International Cloud Atlas (1896) by Hugo Hildebrand Hildebrandsson. Courtesy the UC San Diego Library
In our urge to find patterns we are like a rock climber, pulling herself up the sheer wall of the world. She drives a piton in when she finds a handhold of pattern, a small crevice of meaning, some slight imperfection in the rock face. As a physicist, I believe that this works only because there is already some opening there to grab on to. To say that we construct idealised categories is not to say that patterns in the world don’t already exist, but that we must learn how to see them in the world around us.
Hold up your hand in front of your face: how can you see what’s there? Parsing meaning from randomness – the signal from the noise – is fundamental to both sight and scientific observation. Unless we are blind, our open eyes are flooded with photons at every moment, a ‘noisy’ stream of information that is then launched from the retina, travelling as electrochemical impulses along the optic-nerve pathways. These are taken up by neural assemblies and, in the dark cavern of the skull (filled to the brim as it is with a sloshing assembly of soft matter), the brain sifts that welter of data for ‘signals’ that conform to particular patterns. (Fingers? Check. Five of them? Check.) Some neural assemblies specialise in detecting certain shapes, such as edges or corners; others specialise in collecting those shapes into higher-order schemes, such as a coffee cup, the face of a friend, or your hand.
These internal visual elements are a mix of predilections that we are born with and patterns learned from personal experience; how they affect our perception varies according to our understanding and expectations. When the midcentury psychologists Jerome S Bruner and Leo Postman presented test subjects with brief views of playing cards, including some non-standard varieties – such as a red two of spades, or a black ace of diamonds – many people never called out the incongruities. They reported that they felt uneasy for some reason but often couldn’t identify why, even though it was literally right before their eyes.
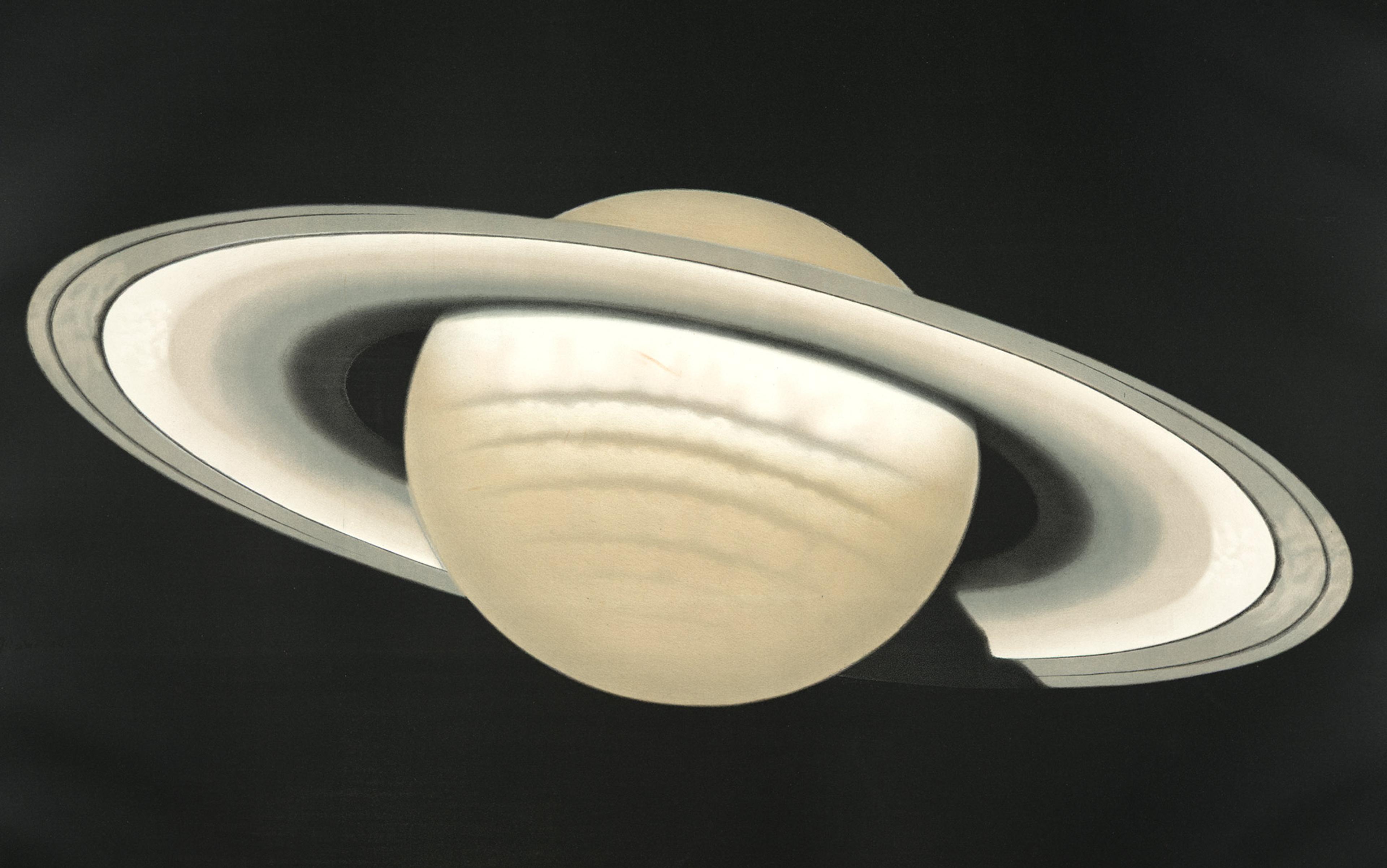
The planet Saturn. Observed on 30 November 1874 at 5.30pm. From the Trouvelot Astronomical Drawings (1882). Courtesy The New York Public Library
So, crucially, some understanding of the expected signal usually exists prior to its detection: to be able to see, we must know what it is we’re looking for, and predict its appearance, which in turn influences the visual experience itself. The process of perception is thus a bit like a Cubist painting, a jumble of personal visual archetypes that the brain enlists from moment to moment to anticipate what our eyes are presenting to us, thereby elaborating a sort of visual theory. Without these patterns we are lost, adrift on a sea of chaos, with a deeply unsettling sense that we don’t know what we are looking at, yet with them we risk seeing only the familiar. How do we learn to see something that is truly new and unexpected?
If the brain is a taxonomising engine, then true learning must always be disorienting
Vision is not only personal and patterned, but also complex and spatially distributed. In the 1970s, Elizabeth K Warrington and Angela M Taylor studied patients with brain damage in the posterior regions of the brain, but with no apparent damage to their visual pathways. It transpired that the part of the brain that’s active when we identify the three-dimensional shape of an object (say, a cylindrical white item on the desk) is different to the area involved in knowing its purpose or name (a coffee cup that holds your next sip). Warrington and Taylor tested subjects’ performance on a ‘same shape’ task by presenting photographs of familiar objects, such as a telephone, but taken from both standard and non-standard perspectives.
For the ‘same function’ task, all the objects were photographed in a standard view but subjects had to group them according to function – for example, to distinguish different sorts of telephones from a mailbox. People with lesions in the right posterior region showed no deficit when objects were presented ‘normally’, but displayed a reduced ability to identify objects when seen from unusual perspectives. Roughly speaking, the results were consistent with the subjects being unable to rotate the object in the mind’s eye into a more ‘standard’ orientation for comparison with internal idealised mental models. Meanwhile, people with lesions in the left posterior brain showed little deficit in such geometric categorisation, yet a reduced ability to identify the object’s name or function.
Subsequent research, now including functional MRI imaging on healthy patients, has led to more nuanced understandings of these matters, but the key insight remains: our extraction of a visual signal is a process distributed across the brain, a silent chorus of the mind that – when it works – produces effortless extraction of visual meaning.
If the brain is a taxonomising engine, anxious to map the things and people we experience into familiar categories, then true learning must always be disorienting. Learning shifts the internal constellation of the firings of our nerves, the star by which we set our course, the spark of thought itself. This mental flexibility is an undeserved inheritance, hard-won over aeons by our ancestors, and it serves as a good metaphor for how scientists can learn to see with new machine eyes.
In science, seeing things afresh sometimes demands a concerted (and contested) shift in paradigms, such as the move from Ptolemy’s map of the planets to those of Copernicus and Galileo. On other occasions, it happens by accident. In a fundamental sense, all of the output of our instruments is signal; noise is just that part we are not interested in. This means that separating out the signal depends upon who is doing the observing and for what purpose. The ‘noise’ picked up by Arno Penzias and Robert Wilson in 1964 in their microwave detectors at Bell Labs in New Jersey turned out to be a clue to one of the most astonishing discoveries in science. After heroic efforts to reduce the background hiss detected by their instrument, they determined that it was not coming from the instrument itself, nor from its suburban surroundings. Instead, they convinced themselves that it was omnidirectional, and coming from the sky. They eventually realised that the hiss was part of the cosmic background, remnant radiation and a ‘signal’ from the Big Bang. Now, 50 years later, the detailed angular distribution across the sky of statistical fluctuations in that radiation is a key piece of evidence that leads many cosmologists to believe in dark energy.
The scientific projects that best stretch our understanding of what it means to see in new ways, and reveal the distributed nature of observation, are those that span the globe and involve thousands of individuals, such as the Laser Interferometer Gravitational-Wave Observatory (LIGO). Efforts such as LIGO are often described as ‘opening a new window’ on the cosmos – yet no human being sees a gravitational wave with LIGO, just as no single neuron in our brain sees a bluebell flower. In both cases, seeing is a multipartite process, requiring a comparison between noisy signals and idealised models.
More than a century ago, Albert Einstein predicted that a warpage of space and time propagates at the speed of light, like the outgoing ripple from the snap of a rug. These space-time ripples are gravitational waves. Consider an incoming burst of gravitational waves, emitted from a distant galaxy by a colliding pair of black holes. Gravity is extraordinarily weak compared with the electromagnetic forces that hold most objects together in our world, giving everyday stuff a solidity that we take for granted. The weakness of gravitational waves therefore means that they have completely negligible effects locally, unless we isolate massive objects from one another and then allow them to move freely in response to the passing space-time ripples. The gravitational waves generate oscillations in the relative positions of these massive objects, not unlike the motions of leaves afloat on the surface of a pond as a ripple passes among them.
Humanity can now see space-time ripples where before we were gravitational-wave blind
If Einstein was right, we are bathed in these ripples but we have been blind to the information they carry – until now. In the case of LIGO, the passage of a gravitational wave produces a shudder – a fraction of a nuclear diameter – in the relative positions of two pairs of 40 kg mirrors, four kilometres apart. Splitting a specially prepared laser beam in two, bouncing it off these mirrors, and allowing the two laser beams to interfere again, the LIGO detector measures a time-varying interference pattern that can be fit to theoretical predictions.
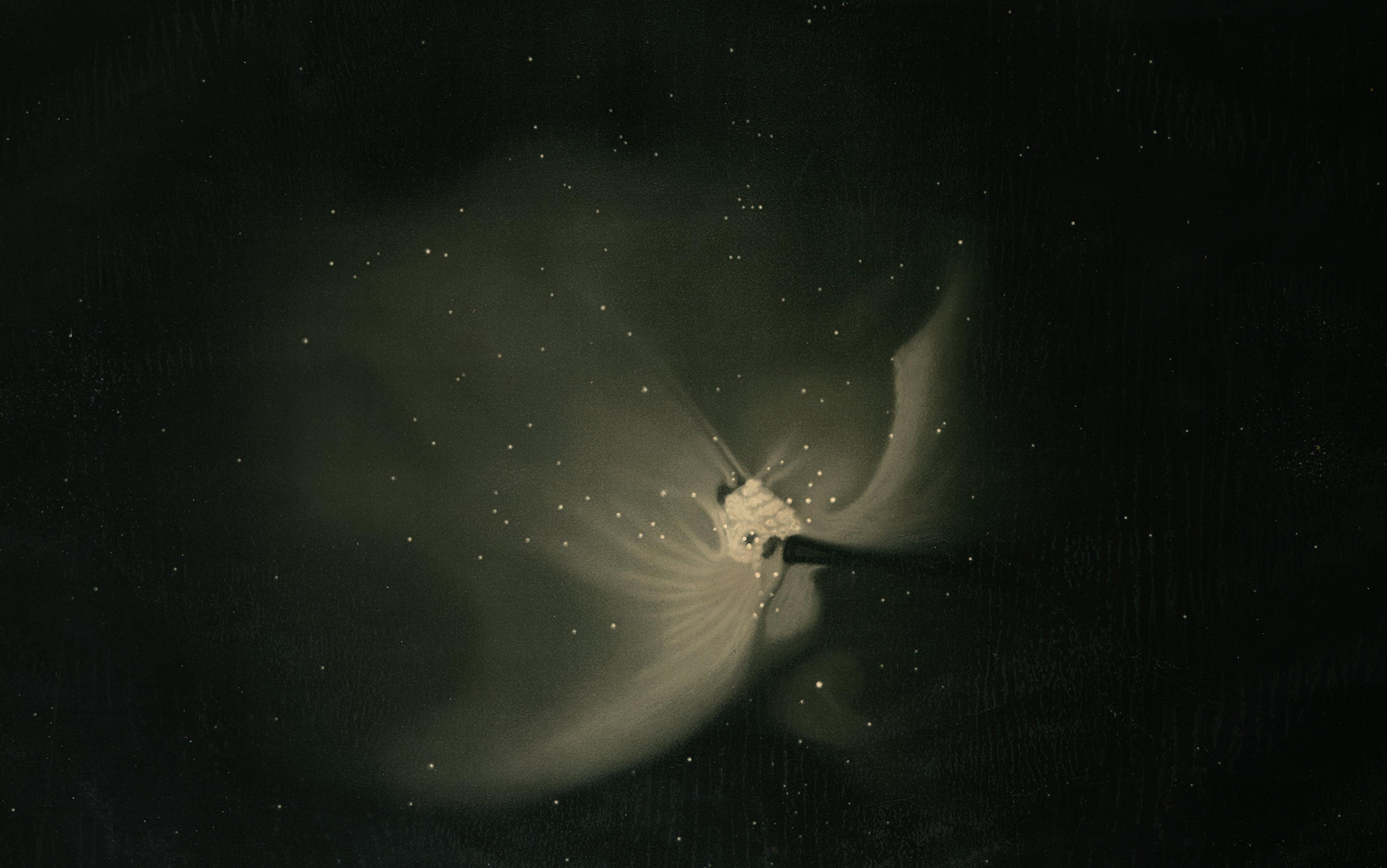
The great nebula in Orion. From a study made in the years 1875-76. From the Trouvelot Astronomical Drawings (1882). Courtesy The New York Public Library
In tandem with the measurement, thousands of simulations are run using the Einstein Equations, the mathematical language of General Relativity. These simulations generate candidate signals – essentially a kind of ‘Gravitational Wave Atlas’ – a numerical compendium of idealisations of what LIGO’s output would look like if certain events had occurred in the distant cosmos. These simulations are answers to ‘What if?’ questions such as: what if two black holes, each 10 solar masses in size, collided 5 billion light-years away? What if one of the black holes was five solar masses, and the other 15, and the distance was 3 billion light-years? By varying the masses and orbital characteristics that initialise the simulation, along with the distance to the event, a best fit to the measured signal is found. If the fit between the measured and idealised model signals is good enough, the LIGO team then infers that two black holes likely collided a billion light-years away. And so, humanity can now see space-time ripples where before we were gravitational-wave blind.
If creating a new telescope, from radio to the gamma-ray, is like adding another electromagnetic eye, LIGO is really like adding an entirely new sense organ. What’s more, our new sense organ can see directly into regions of the Universe that were opaque to all of our previous electromagnetic eyes.
Because of the complexity of both visual experience and scientific observation, it is clear that while seeing might be believing, it is also true that believing affects our understanding of what we see. The filter we bring to sensory experience is commonly known as cognitive bias, but in the context of a scientific observation it is called prior knowledge. To call it prior knowledge does not imply that we are certain it is true, only that we assume it is true in order to get to work making predictions. For example, LIGO researchers assume that the Einstein Equations are universal. This implies that the equations can be tested locally, but they are also assumed to govern the motion of orbiting black holes a billion light-years away.
If we make no prior assumptions, then we have no ground to stand on. The quicksand of radical and unbounded doubt opens beneath our feet and we sink, unable to gain purchase. We remain forever at the base of the sheer rock face of the world, unable to begin our climb. Yet, while we must start with prior knowledge we take as true, we must also remain open to surprise; else we can never learn anything new. In this sense, science is always Janus-headed, like the ancient Roman god of liminal spaces, looking simultaneously to the past and to the future. Learning is essentially about updating our biases, not eliminating them. We always need them to get started, but we also need them to be open to change, otherwise we would be unable to exploit the new vistas that our advancing technology opens to view.
The invention of the telescope heralded a new era of human sight. It led to a flurry of new observations – and great puzzlement. Astronomers were repeatedly confronted with images in their viewfinders that they struggled to make sense of, not only in terms of finding a physical theory for what they were seeing, but something more basic: they struggled to identify stable and repeatable visual patterns, to create a kind of astronomical equivalent to the Cloud Atlas.
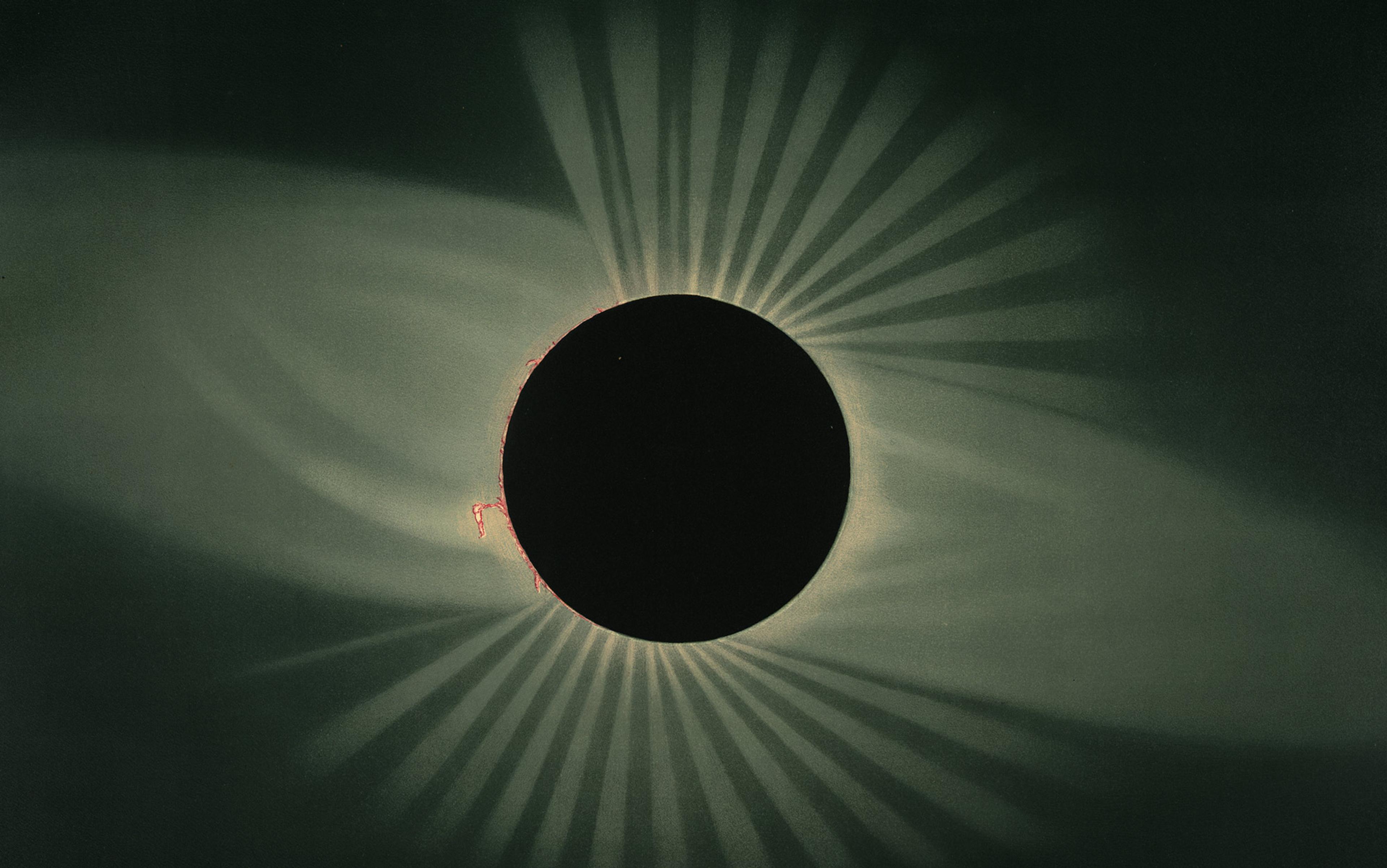
Total eclipse of the sun. Observed 29 July 1878 at Creston, Wyoming Territory. From the Trouvelot Astronomical Drawings (1882). Courtesy The New York Public Library
In Planets and Perception (1988), the astronomy historian William Sheehan notes that a good way to reveal that we don’t understand something is to attempt to draw it. Hence his interest in the early sketches by telescopic observers of the Moon, Mars and the rings of Saturn. What aspects in the visual field were the most essential? What pieces among the collection edges, shapes and textures connected to one another in sensible structures? What was closer, and what was farther away? What was shadowing, and what was colouration? Until Galileo arrived to answer many of these questions, astronomers struggled to interpret their nightly visual experience, and these are the same problems faced today by researchers who are trying to create machine-vision systems.
The iterative bootstrapping of learning-to-see, then seeing-to-learn, continues apace. But in the four centuries since Galileo bent to look through his glazed optic tube, the human brain has not changed all that much, if at all. Rather, the current revolution comes from our new tools, new theories and new methods of analysis made possible by new hardware. Detectors make visible what was previously hidden, and the learning-to-see half of the feedback loop involves ever more powerful and subtle computer algorithms that seek patterns in those new observations. As Daston argues, the objectivity of scientific observation means parsing the world into pieces, and naming those pieces through shared idealisations. But this is now done using a data stream from a global network of detectors and telescopes, aided by smart algorithms to assist in our naming, learning to navigate an information flood that each second dwarfs the amount of data collected by Galileo in a lifetime of observations. Our machines have given us new eyes so we can see things in the world that have been there all along.
If there are frontiers in science, admittedly a fraught metaphor, then we will forever be in the state of the American frontier at the 1890 census – with areas thought roughly settled that are in fact broken up into isolated pockets with immense gaps in between. The evolutionary biologist E O Wilson argues that there are likely still millions of species undiscovered. Where are they? Underfoot, undersea, and lurking in still remote places. We have not plumbed the depths of the natural world, even on our familiar Earth.
If we cast our thoughts outward to the wider solar system and beyond, the mind boggles at how much there is to learn. The world is infinite in all directions, as the theoretical physicist Freeman Dyson wrote in 1988: outward to the stars, inward to the nucleus and, casting sideways, we find the infinite complexity of the biosphere and human cultures, the crenellations and foldings of the human neocortex that somehow contains so much that is light and dark in our beings. An army of scientists will never be enough to grasp the whole of it. That is an open-ended project for the species. Let’s hope that we are always like Galileo setting up his telescope for a night’s viewing, prepared to be astonished, ready to see in new ways, our minds like tinder awaiting a spark.
This essay was made possible through the support of a grant from the Templeton Religion Trust to Aeon. The opinions expressed in this publication are those of the author and do not necessarily reflect the views of the Templeton Religion Trust.
Funders to Aeon Magazine are not involved in editorial decision-making, including commissioning or content-approval.