Biologists like to think of themselves as properly scientific behaviourists, explaining and predicting the ways that proteins, organelles, cells, plants, animals and whole biota behave under various conditions, thanks to the smaller parts of which they are composed. They identify causal mechanisms that reliably execute various functions such as copying DNA, attacking antigens, photosynthesising, discerning temperature gradients, capturing prey, finding their way back to their nests and so forth, but they don’t think that this acknowledgment of functions implicates them in any discredited teleology or imputation of reasons and purposes or understanding to the cells and other parts of the mechanisms they investigate.
But when cognitive science turned its back on behaviourism more than 50 years ago and began dealing with signals and internal maps, goals and expectations, beliefs and desires, biologists were torn. All right, they conceded, people and some animals have minds; their brains are physical minds – not mysterious dualistic minds – processing information and guiding purposeful behaviour; animals without brains, such as sea squirts, don’t have minds, nor do plants or fungi or microbes. They resisted introducing intentional idioms into their theoretical work, except as useful metaphors when teaching or explaining to lay audiences. Genes weren’t really selfish, antibodies weren’t really seeking, cells weren’t really figuring out where they were. These little biological mechanisms weren’t really agents with agendas, even though thinking of them as if they were often led to insights.
We think that this commendable scientific caution has gone too far, putting biologists into a straitjacket that prevents them from exploring the most promising hypotheses, just as behaviourism prevented psychologists from seeing how their subjects’ measurable behaviour could be interpreted as effects of hopes, beliefs, plans, fears, intentions, distractions and so forth. The witty philosopher Sidney Morgenbesser once asked B F Skinner: ‘You think we shouldn’t anthropomorphise people?’– and we’re saying that biologists should chill out and see the virtues of anthropomorphising all sorts of living things. After all, isn’t biology really a kind of reverse engineering of all the parts and processes of living things? Ever since the cybernetics advances of the 1940s and ’50s, engineers have had a robust, practical science of mechanisms with purpose and goal-directedness – without mysticism. We suggest that biologists catch up.
Now, we agree that attributing purpose to objects profligately is a mistake; Isaac Newton’s laws are great for predicting the path of a ball placed at the top of a hill, but they’re useless for understanding what a mouse at the top of a hill will do. So, the other way to make a mistake is to fail to attribute goal-directedness to a system that has it; this kind of teleophobia significantly holds back the ability to predict and control complex systems because it prevents discovery of their most efficient internal controls or pressure points. We reject a simplistic essentialism where humans have ‘real’ goals, and everything else has only metaphorical ‘as if’ goals. Recent advances in basal cognition and related sciences are showing us how to move past this kind of all-or-nothing thinking about the human animal – naturalising human capacities and swapping a naive binary distinction for a continuum of how much agency any system has.
Thanks to Charles Darwin, biology doesn’t ever have to invoke an ‘intelligent designer’ who created all those mechanisms. Evolution by natural selection has done – and is still doing – all that refining and focusing and differentiating work. We’re all just physical mechanisms made of physical mechanisms obeying the laws of physics and chemistry. But there is a profound difference between the ingenious mechanisms designed by human intelligent designers – clocks and motors and computers, for instance – and the mechanisms designed and assembled by natural selection. A simple fantasy will allow us to pinpoint it.
Imagine ordering a remote-controlled model car, and it arrives in a big box that says ‘Some assembly required’ on the back. When you open the box, you find hundreds of different parts, none labelled, and no instruction booklet to help you put the pieces together. A daunting task confronts you, not mainly a problem of the nimbleness or strength of your fingers. Its difficulty lies in not knowing what goes where. A carefully written instruction manual, with diagrams and labels on all the parts would be of great value, of course, but only because you could see the diagrams and read the instructions and labels. If you were sent the Russian instruction manual, it would be almost useless if you didn’t know how to read Russian. (You’d also have to know how to attach Tab A to slot B, and thread nut 17 onto bolt 95.)
But then you spot a slip of paper that instructs you to put all the parts into a large kettle of water on the stove, heat the water to a low boil, and stir. You do this, and to your amazement the parts begin to join together into small and then larger assemblies, with tabs finding their slots, bolts finding their holes, and nuts spinning onto those bolts, all propelled by the random roiling of the boiling water. In a few hours, your model car is assembled and, when you dry it off, it runs smoothly. A preposterous fantasy, of course, but it echoes the ‘miracle’ of life that takes a DNA parts list and instruction book and, without any intelligent assembler’s help, composes a new organism with millions of moving parts, all correctly attached to each other.
We already have a brilliant and detailed account of how the list of ingredients – the genes for proteins – gets read and executed, thanks to those remarkable machines, the ribosomes, the chaperonins and others, and we’re making great progress on how the DNA also provides assembly instructions. Won’t this bottom-up research path, which has been so successful, eventually reveal the details of all the processes that do the work in the imaginary pot of hot water? Is this really a problem?
We think it is. The great progress has been mainly on drilling down to the molecular level, but the higher levels are actually not that well-off. We are still pretty poor at controlling anatomical structure or knowing how to get it back on track in cancer – this is why we don’t have a real regenerative medicine yet. We know how to specify individual cell fates from stem cells, but we’re still far from being able to make complex organs on demand. The few situations where we can make them are those in which we’ve learned to communicate with the cell swarm – providing a simple trigger, such as the bioelectric pattern that says ‘build an eye here’, and then letting the intelligence of the cell group do the hard work and stop when the organ is done.
The more adaptive the agent is to your interference, the more competence it demonstrates
If we stick with the bottom-up approach, all of our bioengineering efforts will come grinding to a halt after the low-hanging fruit (3D-printed bladders and such) are picked. We won’t be micromanaging cell types into a functional human hand or eye in our lifetime; genomic editing approaches won’t have a clue what genes to edit to make complex organs needed for repair or transplantation. Treating cells like dumb bricks to be micromanaged is playing the game with our hands tied behind our backs and will lead to a ‘genomics winter’ if we stay exclusively at this molecular level. The lack of progress in rational morphogenetic control shows us this. For example, despite all the nice papers in Science about the DNA-based regulation of stem cells in planaria, none of the molecular biology models used in these papers makes a prediction on this simple thought experiment:
Take one of the flat-head worms, and implant 50 per cent of the stem cells from a triangular-head worm type. Let the stem cells mix around, and then cut off the head. What shape will we get as it regenerates? What happens when 50 per cent of the stem cells want to build a flat head and the other 50 per cent want to build a triangular head – does one group win? An in-between head shape? Maybe it keeps morphing because some cells are never quite satisfied with the head shape?
The molecular-biology details can’t enable a prediction because they address cell-level questions, and haven’t really touched the issue of how the collective decides what large shape to build and how it decides to stop when that specific shape is complete. The question of representing anatomical target shapes in tissue can be asked experimentally (and it has been) only when one takes seriously that the collective has an information-processing, not just mechanical, level of analysis. This is basically the position that neuroscience was in when David Marr proposed, in his landmark book Vision (1982), that progress would depend on investigating what he called the computational level, by which he meant the level at which one specifies cognitive tasks so that one can then search for the informational processes that could execute those tasks.
Thinking of parts of organisms as agents, detecting opportunities and trying to accomplish missions is risky, but the payoff in insight can be large. Suppose you interfere with a cell or cell assembly during development, moving it or cutting it off from its usual neighbours, to see if it can recover and perform its normal role. Does it know where it is? Does it try to find its neighbours, or perform its usual task wherever it has now landed, or does it find some other work to do? The more adaptive the agent is to your interference, the more competence it demonstrates. When it ‘makes a mistake’, what mistake does it make? Can you ‘trick’ it into acting too early or too late? Such experiments at the tissue and organ level are the counterparts of the thousands of experiments in cognitive science that induce bizarre illusions or distortions or local blindness by inducing pathology, which provide clues about how the ‘magic’ is accomplished, but only if you keep track of what the agents know and want.
Here’s another simple way to think about the problem. Once the individual early cells – stem cells, for instance – are born, they apparently take care of their own further development, shaping both themselves and their local environments without any further instruction from their parents. They become rather autonomous, unlike the mindless gears and pistons in an intelligently designed engine. They find their way. What could possibly explain this? Something like a trail of breadcrumbs? Yes, in some cases, but the cells have to be smart enough to detect and follow them. We might hope for some relatively simple physical explanation.
Here’s another fantasy: it’s all magnets! The parts are moved to their proper homes by, um, thousands of differently tuned varieties of magnetic attraction. The ancient philosopher Thales said that lodestones (natural magnets) have a ‘soul’ that wants iron filings to want to approach them. He was wrong, of course, but could we perhaps account for the apparent strivings and desires of cells by invoking some such deflationary physical forces? Not a chance. No such multidiscriminatory but basic physical forces have been discovered, and there are deep theoretical reasons to conclude that no such forces could exist. What about virtual magnets? We can now make virtual magnets that attract any two things you like. We can make them out of software. In fact, the way that your cursor in Word can stick on its target and the way that highlighting clings to the words in a line are examples of modest virtual magnets that work by passing information around.
It has become standard practice to describe such phenomena with the help of anthropomorphic, intentional idioms: when we click the mouse, we tell the cursor to grab the thing on the screen, and as we move the mouse we move the thing on the screen until we signal to the cursor to drop the thing by clicking the mouse again. This talk of signalling and information-processing is now clearly demystified thanks to computers – no mysterious psychic powers here! – and this has been correctly seen to license use of such information talk everywhere in biology. Detectors and signals and feedback loops and decision-making processes are uncontroversial physical building blocks in biology today, just as they are in computers. But there is a difference that needs to be appreciated, since failure to recognise it is blocking the imagination of theorists. In a phrase that will need careful unpacking, individual cells are not just building blocks, like the basic parts of a ratchet or pump; they have extra competences that turn them into (unthinking) agents that, thanks to information they have on board, can assist in their own assembly into larger structures, and in other large-scale projects that they needn’t understand.
We members of Homo sapiens tend to take the gifts of engineering for granted. For thousands of years, our ancestors prospected for physical regularities that they could exploit by designing structures that could perform specific functions reliably. What makes a good rope, good glue, a good fire-igniter? The humble nut-and-bolt fastener is an elegantly designed exploitation of leverage, flexibility, tensile strength and friction, evolving over 2,000 years, and significantly refined in the past two centuries. Evolution by natural selection has been engaged in the same prospecting at the molecular level for billions of years, and among its discoveries are thousands of molecular tools for cells to use for specific jobs. Among those tools are antennas or hooks with which to exploit the laws of physics and computation.
For example, if you are a cell among some cells that get a sticky protein, you immediately get to make nested spheres, with high-adhesion cells in the middle and low adhesion-cells on the outside. No one has to direct this; it’s a consequence of the laws of physics, but these structured neighbourhoods are inaccessible to you if you don’t have the right proteins to exploit them. If you are part of a multicellular organism and your cells make ferrous particles and arrange them in alignment, suddenly you get to participate in a force that pulls you to Magnetic North. Before, the magnetic field was invisible to you but by having the right proteins arranged in a particular way, bang – you get a whole new competence for free. If you discover an ion channel, suddenly you can participate in all sorts of electrical dynamics. If you get the right kind of ion channels, you immediately get a feedback loop that gives you a bioelectric memory, and other channels give you NOT and AND gates, which enables you to make any kind of Boolean function, giving you ways of exploiting the laws of logic or computation. These are not physical laws but mathematical laws, and you don’t need to understand them to exploit them; all you need is to evolve proteins that allow your subsystems to couple to these dynamics. It’s like the many ways of doing arithmetic: with Roman numerals drawn with a stick in the sand, or Arabic numerals written in pencil on a piece of paper, or beads on an abacus, or flipflops in a computer. Whatever the material medium, if it’s capable of holding results ‘in memory’ while further computation goes on, it can find and use the information detected by the antennas.
The parts themselves must embody the knowhow to find their proper places and do their proper jobs
Notice how ‘you’ can be a single cell or a multicellular organism – or an organ or tissue in a multicellular organism – and still be gifted with informational competences composed out of the basic ‘nuts and bolts’ of information-processing structures. Agents, in this carefully limited perspective, need not be conscious, need not understand, need not have minds, but they do need to be structured to exploit physical regularities that enable them to use information (following the laws of computation) to perform tasks, beginning with the fundamental task of self-preservation, which involves not just providing themselves with the energy needed to wield their tools, but the ability to adjust to their local environments in ways that advance their prospects.
Back to our self-assembling model car. Where does the knowhow to do this reside? In the parts, clearly. Not in the randomly bouncing water molecules, and there’s nobody else in the picture. The parts themselves must somehow embody the knowhow to find their proper places and do their proper jobs. They can be treated as agents with competences that they don’t need to comprehend. Just as Darwin showed how to replace the miraculous intellectual work of the Intelligent Designer with a barely imaginable cascade of trillions of differential replications of organisms that, without knowing or intending anything, gradually designed all the elegant arrangements of nature, so we must now replace the Intelligent Assembler (who would read the instruction manual written by the Intelligent Designer, if there were one) with a bottom-up process that distributes all the requisite intelligence into good design features – competences – at hundreds of levels.
Cone cells on retinas don’t just know how to transduce photons into spike trains; they also know how to get attached to the right neurons to put them into indirect contact with the neural nets that accomplish vision in sighted organisms. But unlike the intelligent assembler who, from reading the instruction book, knows how to wire the model car’s headlights in the front to the battery in the back, the cone cell’s knowledge is myopic in the extreme. Its Umwelt is microscopic. It’s an idiot savant, with some amazing talents but no insight at all into those talents. But thanks to communication – signalling – with its neighbours, it can contribute its own local competence to a distributed system that does have long-range information-guided abilities, long-range in both space and time.
Yet there seems to be a fundamental problem here. Evolution runs on the principle of selfishness. How could complex living systems implement group goals toward which their cellular subunits would work? How can such cooperation ever arise from the actions of selfish reproducing agents? Consider the thinking tool that has been used to analyse this conundrum for decades – the Prisoner’s Dilemma (PD) – as set out in Daniel Dennett’s book Darwin’s Dangerous Idea (1995):
The best-known example in game theory is the the Prisoner’s Dilemma, a simple two-person ‘game’ [in which] you and another person have been imprisoned pending trial (on a trumped-up charge, let’s say), and the prosecutor offers each of you, separately, the same deal: if you both hang tough, neither confessing nor implicating the other, you will each get a short sentence (the state’s evidence is not that strong); if you confess and implicate the other and he hangs tough, you go scot free and he gets life in prison; if you both confess-and-implicate, you both get medium-length sentences. Of course, if you hang tough and the other person confesses, he goes free and you get life. What should you do?
If you both could hang tough, this would be much better for the two of you than if you both confess, so couldn’t you just promise each other to hang tough? (In the standard jargon of the Prisoner’s Dilemma, the hang-tough option is called cooperating.) You could promise, but you would each then feel the temptation – whether or not you acted on it – to defect, since then you would go scot-free, leaving the sucker, sad to say, in deep trouble. Since the game is symmetrical, the other person will be just as tempted, of course, to make a sucker of you by defecting. Can you risk life in prison on the other person’s keeping his promise? Probably safer to defect, isn’t it? That way, you definitely avoid the worst outcome of all, and might even go free. Of course, the other fellow will figure this out, too, so he’ll probably play it safe and defect as well, in which case you must defect to avoid calamity – unless you are so saintly that you don’t mind spending your life in prison to save a promise-breaker! – so you’ll both wind up with medium-length sentences. If only you could overcome this reasoning and cooperate!
Specifically, let’s think about spatialised PD, with a grid of agents (cells) and each one plays PD against its neighbours. The traditional way to think about this is that the number of agents is fixed – they are permanently distinct, and the only thing that varies and evolves is the policy that each one uses to decide to cooperate or defect against its neighbours. But imagine that evolution discovers a special protein – a Connexin – that allows two neighbouring cells to directly connect their internal milieus via a kind of tunnel through which small molecules can go. Evolution’s discovery of this kind of protein allows the system to take advantage of a remarkable dynamic.
When two cells connect their innards, this ensures that nutrients, information signals, poisons, etc are rapidly and equally shared. Crucially, this merging implements a kind of immediate ‘karma’: whatever happens to one side of the compound agent, good or bad, rapidly affects the other side. Under these conditions, one side can’t fool the other or ignore its messages, and it’s absolutely maladaptive for one side to do anything bad to the other because they now share the slings and fortunes of life. Perfect cooperation is ensured by the impossibility of cheating and erasure of boundaries between the agents. The key here is that cooperation doesn’t require any decrease of selfishness. The agents are just as 100 per cent selfish as before; agents always look out for Number One, but the boundaries of Number One, the self that they defend at all costs, have radically expanded – perhaps to an entire tissue or organ scale.
Planaria are champion regenerators, making exactly the same anatomy every time they are cut
This physiological networking fundamentally erases the boundaries between smaller agents, forming a kind of superagent in which the individual identity of the original ones is very hard to maintain. Of course, these boundaries aren’t anatomical, they’re physiological or functional – they demarcate computational compartments inside of which data flow freely, with massive implications from a game-theory perspective. Information (memory) is now shared by the collective – indeed, that is a prime reason for connecting to your neighbour: you inherit, for free, the benefit of their learning and past history for which they already paid in metabolic effort.
The other amazing thing that happens when cells connect their internal signalling networks is that the physiological setpoints that serve as primitive goals in cellular homeostatic loops, and the measurement processes that detect deviations from the correct range, are both scaled up. In large cell collectives, these are scaled massively in both space (to a tissue- or organ-scale) and time (larger memory and anticipation capabilities, because the combined network of many cells has hugely more computational capacity than the sum of individual cells’ abilities). This means that their goals – the physicochemical states that serve as attractors in their state space – are also scaled up from the tiny, physiological homeostatic goals of single cells to the much larger, anatomical homeostasis of regeneration and development, which can achieve the correct target morphology from all kinds of weird starting configurations, and despite noise and massive external perturbations along the way (see Figure 1 below). It is this system that later became coopted to the even larger and faster goal-directed activity of brains, as ancient electrical synapses (Connexin-based gap junctions) evolved into modern chemical synapses, and cells (which were using physiological signalling to orchestrate morphogenesis) became speed-optimised lanky neurons.
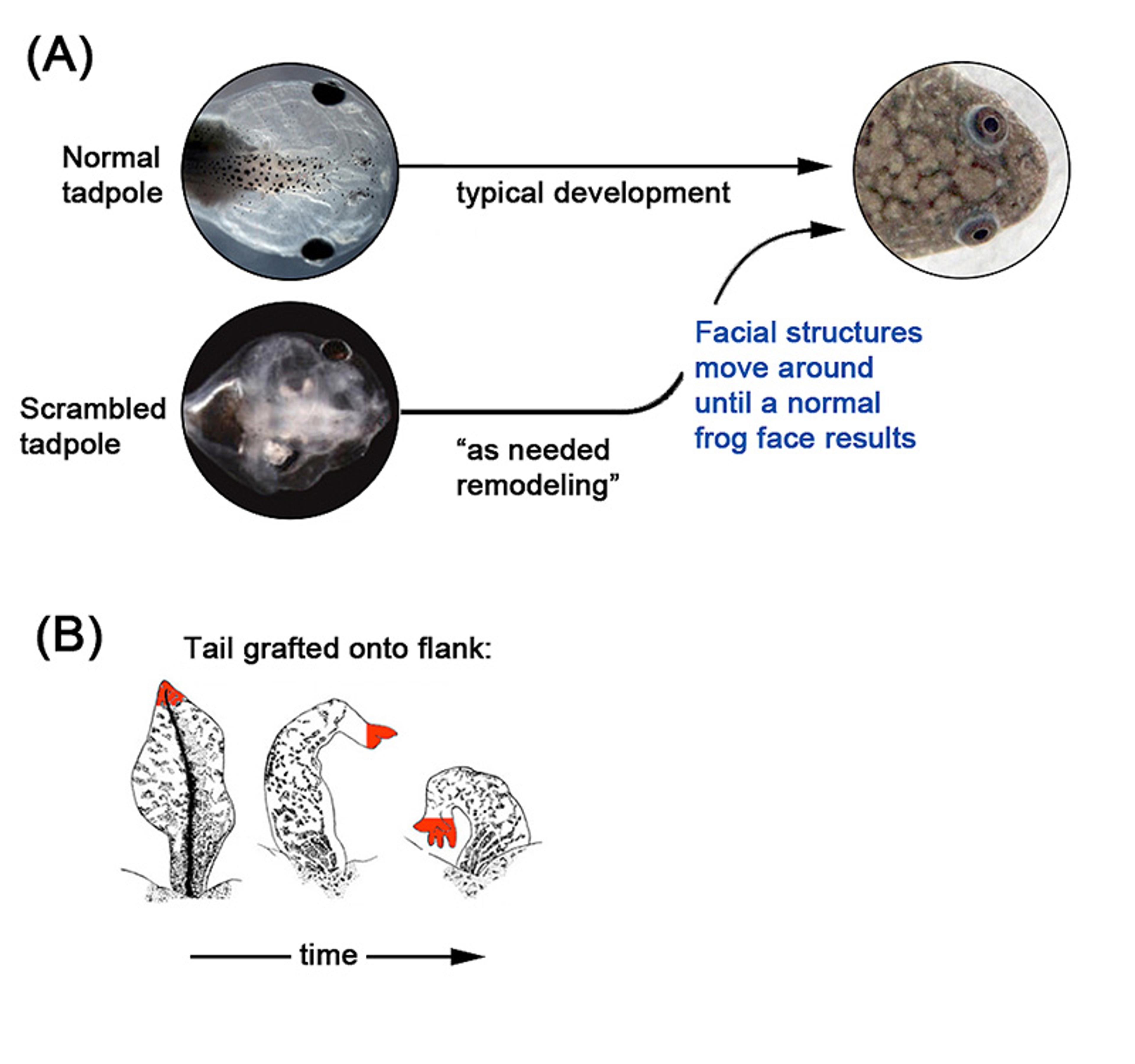
Figure 1: Cells flexibly cooperate toward specific complex anatomies, such as organs in the tadpole face that rearrange if placed in unnatural positions to make a normal frog face, or salamander tails grafted to the flank of the animal that turn into the more appropriate structure – limbs. Images in panel A (normal tadpole) are courtesy of Douglas J Blackiston, Levin lab and scrambled tadpole image is courtesy Vandenburg et al. Images in panel B are from Farinella-Ferruzza 1956
The cooperation problem and the problem of the origin of unified minds embodied in a swarm (of cells, of ants, etc) are highly related. The key dynamic that evolution discovered is a special kind of communication allowing privileged access of agents to the same information pool, which in turn made it possible to scale selves. This kickstarted the continuum of increasing agency. This even has medical implications: preventing this physiological communication within the body – by shutting down gap junctions or simply inserting pieces of plastic between tissues – initiates cancer, a localised reversion to an ancient, unicellular state in which the boundary of the self is just the surface of a single cell and the rest of the body is just ‘environment’ from its perspective, to be exploited selfishly. And we now know that artificially forcing cells back into bioelectrical connection with their neighbours can normalise such cancer cells, pushing them back into the collective goal of tissue upkeep and maintenance.
An important implication of this view is that cooperation is less about genetic relatedness and much more about physiological interoperability. As long as the hardware is good enough to enable physiological communication of this type, the exact details aren’t nearly as important as the multiscale homeostatic dynamics ensured by the laws of physics and computation. For example, unlike us humans, whose children never inherit mutations occurring in our bodies during our lifetime, planarian flatworms often reproduce by fission and regeneration. This means that they have somatic inheritance – every mutation that doesn’t kill the stem cell is propagated into the next generations as the stem cell reproduces to help fill in the missing half of the worm. Their genomes bear the evidence of this accumulation of junk over 400 million years – they are a total mess. We still don’t have a proper genome assembly for Dugesia japonica, and the worms are mixoploid – they don’t even all have the same number of chromosomes! And yet, planaria are champion regenerators, making exactly the same anatomy every time they are cut – 100 per cent fidelity, despite the mess of a genome. This is what computer scientists call hardware independence – within a wide tolerance, the same worm-building software can run on very different molecular hardware because the software is extremely robust and directed to build the same large-scale outcome whatever it takes (in terms of telling the diverse cells what to do to get there).
How do the ‘right’ cells ‘figure out’ whom they should trust as a life partner? We don’t see every cell partnering up with every other cell that is its neighbour. So the Prisoner’s Dilemma still seems to raise its head. Is there some sort of trial-and-error Darwinian process that homes in on the best gap junctions to commit to? How can the individual cells tell which way to turn? At the cellular level, most cells can couple with most other cells. What determines how this happens is the physiological software, which determines whether gap junctions between any two cells are open or not. Remarkably, the physiological software isn’t ‘hardwired’ or even ‘firmware’; these gap junctions have – like synapses – a memory, and are affected by prior states of the cells.
Indeed, because they are voltage-gated current conductors, they are in effect transistors; and we already know how powerful transistors are as a basic element of memory, feedback loops (basic homeostasis or amplification), and coordinated decision-making. Cells have policies for joining up with other cells based on sharing nutrients and diluting toxins (a trick learned from the protobodies of bacterial biofilms), as well as sharing information that enables better predictions and thus homeostatic success. In fact, they even gang up on dissenters in a process known as normalisation – any cell that is physiologically aberrant will experience the neighbours’ trying to bring it into the large-scale physiological plan by its neighbour cells simply trying to average its voltage (for example) via the gap-junctional connections.
This is a reasonable mechanistic story, but then isn’t all the talk of memory, decision-making, preferences and goal-driven behaviour just anthropomorphism? Many will want to maintain that real cognition is what brains do, and what happens in biochemistry only seems like it’s doing similar things. We propose an inversion of this familiar idea; the point is not to anthropomorphise morphogenesis – the point is to naturalise cognition. There is nothing magic that humans (or other smart animals) do that doesn’t have a phylogenetic history. Taking evolution seriously means asking what cognition looked like all the way back. Modern data in the field of basal cognition makes it impossible to maintain an artificial dichotomy of ‘real’ and ‘as-if’ cognition. There is one continuum along which all living systems (and many nonliving ones) can be placed, with respect to how much thinking they can do.
Tissues, organs, brains, animals and swarms form various kinds of minds that can reach for bigger goals
You have to remember that, while the most popular stories about how cells cooperate toward huge goals are about neural cells, there is little fundamental difference between neurons and other cell types. It is now known that synaptic proteins, ion channels and gap junctions, for instance, were already present in our unicellular ancestors, and were being used by electrically active cells to coordinate actions in anatomical morphospace (remodelling and development) long before they were co-opted to manage faster activity in 3D space. If you agree that there is some mechanism by which electrically active cells can represent past memories, future counterfactuals and large-scale goals, there is no reason why non-neural electric networks wouldn’t be doing a simplified version of the same thing to accomplish anatomical homeostasis. Phylogenetics has made it very clear that neurons evolved from far simpler cell types, and that some of the brain’s speed-optimised tricks were discovered around the time of bacterial biofilms (the biggest trick being scaling up into networks that can represent progressively bigger goal states and coordinating the Test-Operate-Test-Exit loop across tissues). Cognition has been a slow climb, not a magical leap, along this path.
The central point about cognitive systems, no matter their material implementation (including animals, cells, synthetic life forms, AI, and possible alien life) is what they know how to detect, represent as memories, anticipate, decide among and – crucially – attempt to affect. Call this the system’s cognitive horizon. One way to categorise and compare cognitive systems, whether artificial or evolved, simple or complex, is by mapping the size and shape of the goals it can support (represent and work toward). Each agent’s mind comprises a kind of shape in a virtual space of possible past and future events. The spatial extent of this shape is determined by how far away the agent can sense and exert actions – does it know, and act to control, events within 1 cm distance, or metres, or miles away? The temporal dimension is set by how far back it can remember, and how far forward it can anticipate – can it work towards things that will happen minutes from now, days from now, or decades from now?
Humans, of course, have very large cognitive horizons, sometimes working hard for things that will happen long after they are gone, in places far away. Worms work only for very local, immediate goals. Other agents, natural and artificial, can be anywhere in between. This way of plotting any system’s cognitive horizon is a kind of space-time diagram analogous to the ways in which relativistic physics represents an observer’s light cone – fundamental limits on what any observer can interact with, via influence or information. Examples are shown in Figure 2 below. It’s all about goals: single cells’ homeostatic goals are roughly the size of one cell, and have limited memory and anticipation capacity. Tissues, organs, brains, animals and swarms (like anthills) form various kinds of minds that can represent, remember and reach for bigger goals. This conceptual scheme enables us to look past irrelevant details of the materials or backstory of their construction, and to focus on what’s important for being a cognitive agent with some degree of sophistication: the scale of its goals. Agents can combine into networks, scaling their tiny, local goals into more grandiose ones belonging to a larger, unified self. And of course, any cognitive agent can be made up of smaller agents, each with their own limits on the size and complexity of what they’re working towards.
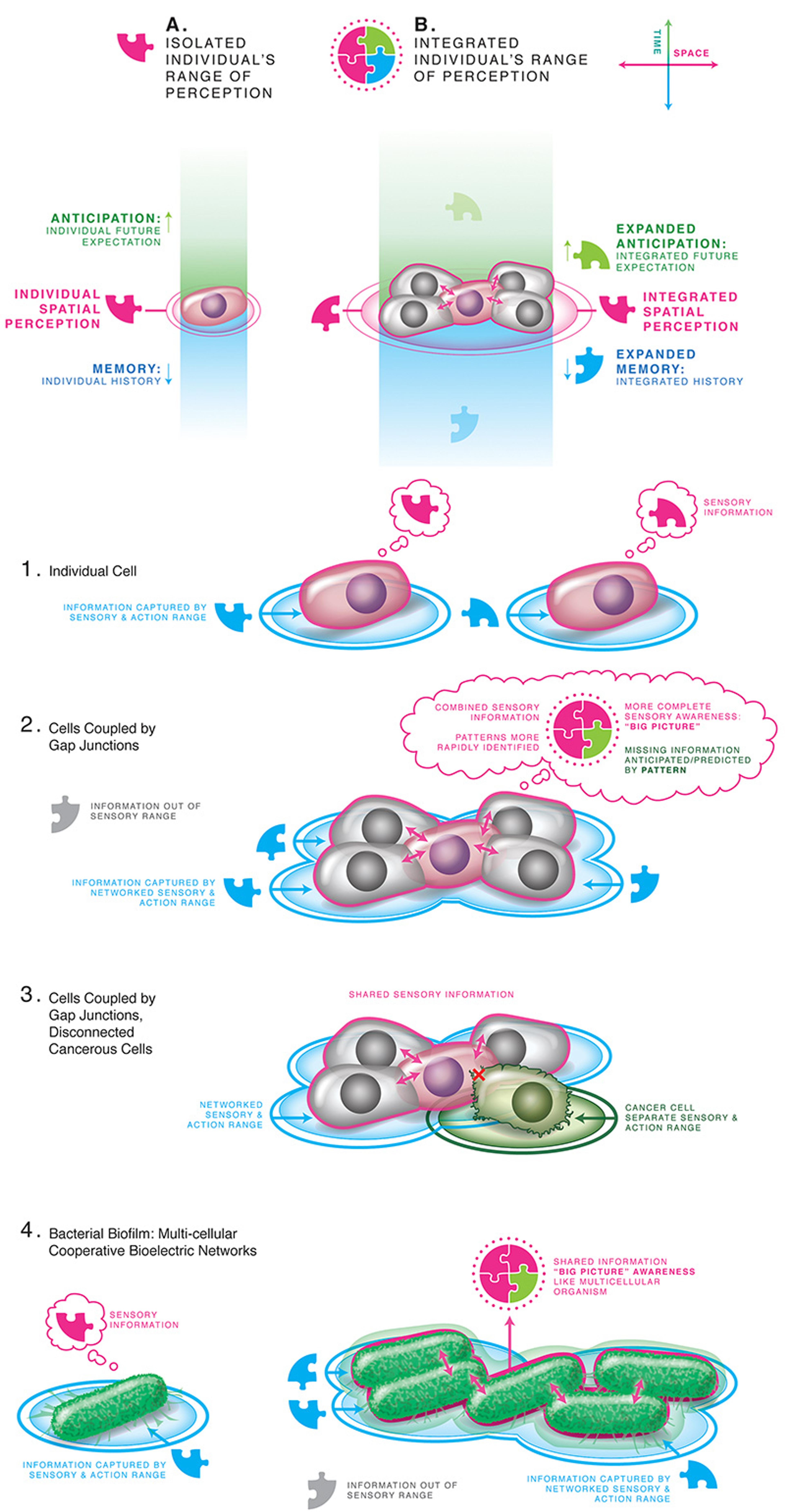
Figure 2: The cognitive boundary of a self can expand from the small spatial and temporal scale of single-cell physiological homeostasis to the much larger anatomical goals of organogenesis, by linking cells via bioelectrical synapses into a single coherent system. Graphics drawn by Jeremy Guay of Peregrine Creative Inc
From this perspective, we can visualise the tiny cognitive contribution of a single cell to the cognitive projects and talents of a lone human scout exploring new territory, but also to the scout’s tribe, which provided much education and support, thanks to language, and eventually to a team of scientists and other thinkers who pool their knowhow to explore, thanks to new tools, the whole cosmos and even the abstract spaces of mathematics, poetry and music. Instead of treating human ‘genius’ as a sort of black box made of magical smartstuff, we can reinterpret it as an explosive expansion of the bag of mechanical-but-cognitive tricks discovered by natural selection over billions of years. By distributing the intelligence over time – aeons of evolution, and years of learning and development, and milliseconds of computation – and space – not just smart brains and smart neurons but smart tissues and cells and proofreading enzymes and ribosomes – the mysteries of life can be unified in a single breathtaking vision.